Data Quality Tools
Understanding Data Quality
Data quality ensures that the data available to the entire organization is clean and manageable. The core of data-driven decisions comes from the quality and authenticity of the data set. The data is first collected, then filtered, and then processed to extract the useful context to make viable decisions. Nowadays, all companies are striving to base their important decisions on data. This is because data-driven decision making provides practical reasoning backed-up by validated numbers and statistics. To make sure your company is grounding its decision free from all biases and inaccuracies, it is the best idea to use data science techniques to utilize data quality enhancement tools.
According to recent trends, data quality can easily be regarded as the company’s biggest asset. As blood moves throughout the body and keeps it alive, data flows in the entire organization and keeps it working well. Typically, the input is from multiple data sources and each one has several layers of complexities. Data quality tools work as regulatory bodies that police the authenticity and reliability of data so that the extracted information can be reused.
Data quality management tools preserve the business-readiness of the data in the entire data pipeline.
By implementing the DQM solution by BiG EVAL, your firm will enhance its data integrity and get the best out of your data.
Understanding Data Quality Tools
Nowadays, almost any process can be automated. Similarly, the scripts that help maintain data quality are called data quality tools. These tools heavily rely on identification, understanding, and rectification of data flaws and inconsistencies. This helps to upkeep with good data governance all across the data-driven cycle which involves business and process decisions. The common functions that all good data quality management tools must perform are;
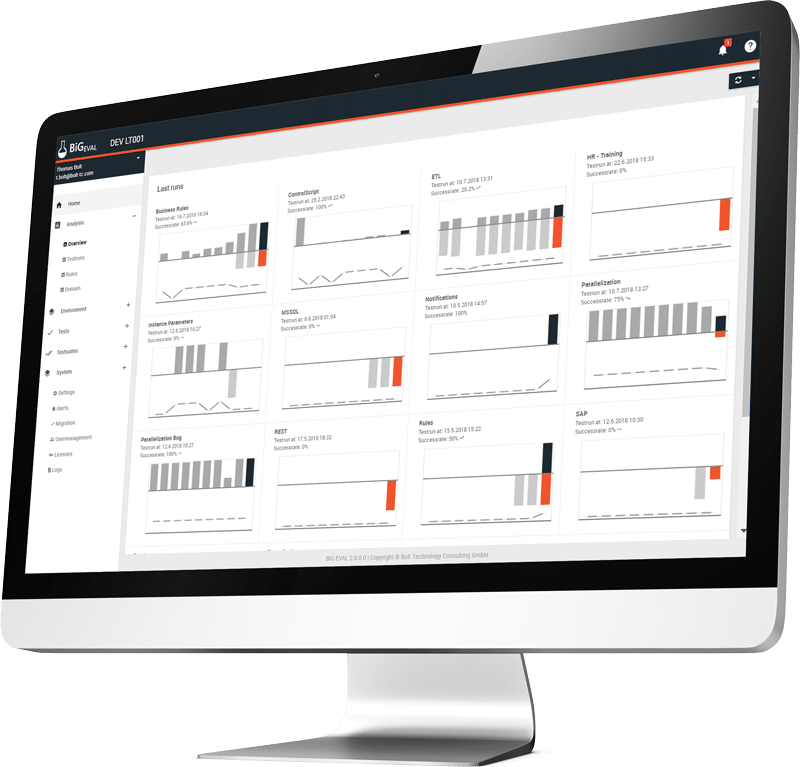
Firms have a lot of information these days. To make the best out of the data in hand, companies have dedicated data analytics teams. Newly hit computer and data science terminologies such as cloud computing, big data, and data-driven decisions are all linked. Data-driven means to base important decisions, which may affect the revenue, on the underlying information within the data.
Data analysts are professional “meaning hunters” who find meanings, and patterns in the data sets for businesses to make financially sound decisions. Such decisions are called data-driven decisions making the best use of data science technologies. So maintaining high data quality is necessary.
Selecting the Right Data Quality Tool
With the advent of so many tools and techniques, it is a crucial task to identify what you require exactly. Selecting the right data quality tool is important and impacts the final results in hand. There are a few considerations that one has to make the correct selection.
Identification of data challenges that you are facing.
There are various types of data challenges a firm might face. It could be the problem of incorrect data, missing data, duplicate data, and many more data integrity issues. All these lower the success rate of the business. Maintaining data integrity is critical and it cannot be ignored. So data challenges must be correctly identified. Improved data quality can ensure the right effort is put in the right direction and employees are well utilized. Analysis of current data sources and existing tools can make the existing situation prominent and problems can be troubleshot earlier.
Your Benefits:
BiG EVAL stands out by the following characteristics:
- Easy and understandable user interface with a high practice orientation. This ensures an easy introduction with a steep learning curve.
- Robust support of your data quality processes with integration of business experts.
- Seamless combination of test automation during system development and data quality management in productive environments.
- Great Cost/Performance Ratio.
- Data Quality Software delivers the desired results within seconds.
- Industry-wide confidentiality.
Identification of scope of data quality tools – what they can and cannot do.
Every data quality tool has some limitations. It cannot magically fix an absurdly ill data source. The worst data are those which are broken, illogically joint, outdated, and sloppy spreadsheets. These occur mainly because of wrong data collection and gathering techniques. Data quality tools cannot reverse the effect of wrong data collection techniques completely. In such cases, it is important that initial processes are redesigned and data frameworks are rewritten. A tool like BiG EVAL Data Test Automation can help you implementing these technical processes error free. With improved organizational techniques to store, gather and manage the workflow of data, data quality tools can work for you.
Identification of your data cleansing requirements.
Enterprise data can get complicated. To select the right tool, data in hand needs to be examined and data cleansing requirements have to be well thought out. After successfully doing so, it can be decided what to do next and which tool to use.
Identification of strengths and weaknesses of the desired tool.
Lastly, all tools are not the same. Each has its own strengths and specific functionality. Some are made for certain types of applications such as SAP, Salesforce, and others are made of Spreadsheets, and some are for data parsing tools. It is also important to note that each tool has different security, licensing, and package details. Select the one which suits your needs the most.
Understanding the Inabilities of Standalone Data Quality Tools
The market is filled with various standalone data quality tools. They claim to clean bad data, but all that is actually done as haphazard cleaning. In the short run, these standalone tools can make the illusion of being helpful. But in the grand scheme of data quality management, they are of little help. A good data quality tool needs easy deployment whereas these standalone tools require complex deployment, custom settings, and deep expertise. All in all, a quick fix can be disastrous in the longer run.Understanding Integrative Data Quality Building Approach
Data quality management has many faces. One of which is a proactive approach where data quality is measured and checked at the earlier stages of the data lifecycle. This eradicates the data issues before it even enters the stream of the system. Then it is important to keep measuring the data across all grounds; may it be in the cloud, web, or even internal. This is not an easy set of tasks. The only way to make this continuous monitoring happen is by enabling data integration. As there are real-time sets of data, data quality management tools come in handy. This also ensures that when errors were detected at early stages, the respective piece of information is not carried forward to later stages. The right combination of data quality management tool and integrated data approach can do wonders to eradicate the root cause of most of your data integrity problems and data gaps, simultaneously ensuring smooth data-driven lifecycle and decision making at a firm.Data moves across the enterprise’s products and systems so it has to be in a standardized form. Firms can have their own standards. The activities of data parsing, standardization, and matching data with real-time incoming data feeds take place as a result. One shortcut method adopt to lessen the heftiness of this task is to miss collaborative data management. This means how various data and data quality tools understand each other’s results. This is a challenge as we discussed in the standalone approach where we came to the conclusion, that using a singular solution is not the right approach. Enterprises need to acquire platform-based solutions which operate, share and interlink their findings easily. BiG EVAL DQM for example is able to exchange data quality metrics and detailed results with many other tools. In special case it is even able to run custom code to push or pull information to other systems.
Shifting Data Quality Tools into the Cloud
Enterprises are building own teams to manage their data successfully. To facilitate the operations and teams, it makes sense to move data quality practices into the cloud where they are present at all times even on an ad hoc basis. This will not only empower the data quality management team but also the managers of other data teams as collaboration is much easier.Shifting to cloud-based technologies makes teams more efficient around the whole globe. DQM teams give the feedback, that handling traditional issues such as data cleansing, data reconciliation, data matching, and resolution are becoming much easier when DQM tools are based on the cloud.
Cloud DQM techniques rely on the following;
Data Profiling
It is the process of determining the type, shape and condition of stored data. It is a vital step that enables control over the data. This enables complete visibility of details and all records. Custom business logic and statistical profiling of the data are then successfully implemented.
Data Stewardship
It is the process of handling the lifecycle of data from step one of curation till the last step of retirement. It defines data models on data that can be applied. This step ensures that correct data governance can be applied to data sets including data monitoring, reconciliation, data refining, redundancy removal, data cleansing, and data aggregation. This helps delivering better data quality to the end-users.
Data Preparation
It is the process of enriching the data. Data-driven organizations rely on preparation utilities that provide self-service capabilities to professionals. Data experts are looking for tools that can portray the data in the best form possible.
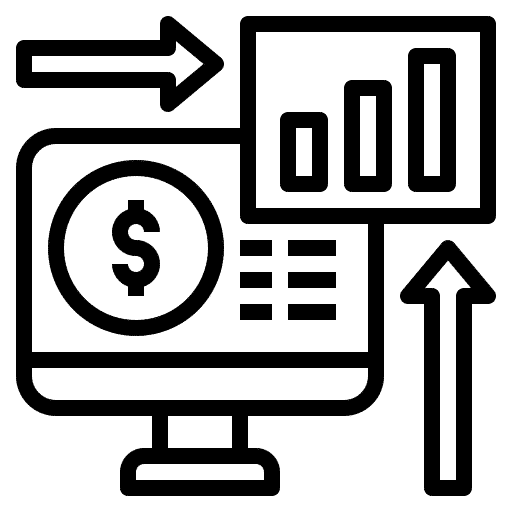
Common DQM tools available in the market
- BiG EVAL
- Cloudingo
- Data Ladder
- IBM’s InfoSphere & QualityStage
- Informatica’s Master Data Management
- OpenRefine
- SAS’s Data Management
- Syncsort Trillium
- Talend’s Data Quality
- TIBCO’s Clarity
- Validity’s DemandTools
- BiG EVAL’s DQM solution provides a combination of all advantages.
Comparing Data Quality tools
The main aspect which defines which tool to opt for is its focus. Some focus on salesforce data such as Cloudingo, and some focus on big data such as IBM. All have a distinct feature which enterprises study to select the best one. Organizations select the one which best suits their needs.Summing up, Data quality management is a critical step to enable organizations to use true data-driven models and automated data quality tools are the best way to achieve good data quality in an efficient way.
To be successful means to be able to handle existing data correctly, efficient and sustainable.
BLOG