The Data Quality Business Case
How to Get Budget and Executive Buy-In to Improve Data Quality
Are your data quality KPIs failing to impress management? Does it feel like you're speaking a different language when asking for data quality improvement budgets? Discover the key to translating data quality into the language of business – dollars and cents. This guide will help you build a persuasive case that highlights the true financial value of data quality initiatives.
Introduction
The Hidden Cost of Poor Data Quality
Imagine this: Your marketing team launches a highly targeted campaign based on seemingly accurate customer data. After weeks and a significant budget investment, the results are in, and they're dismal. Upon deeper investigation, you discover a large portion of your customer information contained duplicate records, outdated addresses, and missing fields. The campaign was doomed from the start due to poor data quality.
This scenario is far more common than many organizations realize. Gartner estimates that the average company loses $12.9 million per year due to bad data. The consequences go beyond wasted marketing costs– poor data quality can hinder sales performance, slow down operations, damage your reputation, and even expose you to regulatory risks.
The Problem with Focusing on Technical Metrics
Data professionals know the importance of metrics like accuracy, completeness, consistency, and timeliness. These data quality key performance indicators (KPIs) are essential tools for monitoring and maintaining data health. However, when it comes to convincing management to invest in data quality initiatives, technical KPIs often fall flat. Why? Because executives think in terms of revenue, costs, customer satisfaction, and risk. While technical metrics are essential for the data team, they don't directly translate into the language that resonates with those who hold the purse strings.
The Importance of Data Quality for Business Success
The Role of Data in Decision-Making
Data-driven decision-making separates leaders from those lagging behind. The quality of your data directly impacts the quality of your decisions. Incorrect data, inconsistencies, or missing information can lead to:
- Misguided marketing investments: Targeting the wrong audience, offering irrelevant promotions, or wasting resources on dead-end leads.
- Inefficient operational processes: Bottlenecks caused by manual error correction, delays in order processing, and inaccurate inventory management.
- Flawed customer segmentation strategies: Misunderstanding customer needs, resulting in ineffective personalization or poor product recommendations.
- Unreliable sales forecasts: Inaccurate projections leading to overstocking/understocking, inefficient resource allocation, and missed revenue targets.
- Compliance risks and potential fines: Failure to comply with regulations like GDPR, CCPA, or industry-specific standards due to poor data management.
How Data Quality Impacts the Bottom Line
Poor data quality erodes profits in both visible and hidden ways. Direct costs include wasted resources, rework, and lost opportunities. Indirect costs are even more damaging – impaired customer trust, tarnished brand reputation, and hampered innovation.
Data Quality for Decision-Makers: Why Business Value Matters More Than Technical KPIs
Understanding the Language of Business
For CIOs, project managers, and anyone needing to secure a data quality budget, mastering the language of business is crucial. While technical KPIs offer value to data specialists, they don't resonate with decision-makers. To unlock funding, you need to speak in terms of:
- Increased revenue: How can better data quality lead to more effective sales and marketing, identifying new prospects, improving conversion rates, or boosting customer lifetime value?
- Reduced costs: Where can data quality improvements streamline operations, cut down on rework, reduce waste, and optimize resource utilization?
- Improved customer experience: How does high-quality data enhance personalization, reduce errors during transactions, provide faster issue resolution, and ultimately boost customer satisfaction and retention?
- Mitigated risk: How can data quality help avoid regulatory fines, protect against reputational damage due to data breaches or misuse, and ensure compliance?
Translating Data Quality into Financial Impact
Words like "accuracy" and "completeness" are abstract to non-data specialists. To truly secure management buy-in, you need to put a price tag on the costs of bad data, and the potential gains from quality enhancements.
Building a Compelling Data Quality Business Case
Step 1: Identify Business Pain Points
Don't start with the technology; start with the problems your organization faces. Interview stakeholders from various departments to understand their specific challenges:
- Sales: Are leads lost due to inaccurate contact information? Are sales cycles longer due to time spent fixing data errors? Does poor data quality hinder cross-selling and up-selling opportunities?
- Marketing: Are campaigns underperforming due to flawed audience targeting? Is personalization hampered by incomplete customer profiles? Can marketing ROI be accurately calculated?
- Operations: Are there bottlenecks caused by manual data cleansing or error correction? Are production delays caused by inaccurate inventory management or supply chain data?
- Customer service: Do agents face long resolution times due to fragmented or duplicate customer data? Are customers frustrated by repeated requests for information or the inability to track orders correctly?
- Finance: Is financial reporting delayed or inaccurate due to reconciliation errors caused by inconsistent data? Are there compliance risks due to poor data governance in financial transactions?
- IT: Are many hours spent in fixing data quality issues manually? Are there many frustrating fire fighting drills to bring data systems back running error-free?
Step 2: Quantify the Cost of Bad Data
Once you've identified pain points, work to analyze the financial impact. Consider:
- Lost revenue: Calculate missed sales opportunities due to incorrect data. For example, if your average order value is $100, and inaccurate contact information leads to 50 lost leads per month, that equates to $5,000 in potential lost revenue.
- Wasted resources: Estimate the time and costs spent on manual data fixing, rework, and additional customer support. If an employee spends two hours per day on error correction at an average hourly rate of $30, it amounts to $360 per week in wasted resources.
- Compliance risks: Look at past fines or estimate the potential cost of non-compliance. GDPR violations can reach up to €20 million or 4% of annual global revenue. Even if you haven't faced fines yet, consider the cost of legal counsel and remediation in the event of a data breach.
- Reputation damage: While hard to quantify directly, consider the long-term impact on customer loyalty and market perception caused by repeated errors, frustrating customer experiences, and data breaches.
Step 3: Project the Benefits of Data Quality Improvement
Now outline how improving data quality will address these pain points. Be specific:
- Increased revenue: If improved lead data boosts conversions by 10%, how much additional revenue is generated annually? If accurate inventory data reduces stockouts by 5%, what's the gained sales value?
- Reduced costs: If streamlined processes save 20 hours of employee time per week, calculate the annual cost savings. Project savings in reduced support tickets from resolving data errors.
- Improved customer experience: How might faster issue resolution and personalized offers impact customer satisfaction scores (e.g., NPS)? Link those to potential revenue gains from repeat business and positive referrals.
- Mitigated risk: If data quality compliance avoids potential regulatory fines, include those amounts. Consider reduced insurance costs due to improved data security and lowered risk of data breaches.
Step 4: Develop Your Solution Roadmap
Outline the technologies, processes, and resources needed to improve data quality. This shouldn't be an in-depth technical plan but rather a high-level roadmap demonstrating that you have a clear strategy:
- Data cleansing and standardization: Tools and processes to fix existing errors and create rules for maintaining consistency.
- Data validation and monitoring: Solutions to analyze data health regularly and flag potential issues.
- Data governance framework: Clear policies, roles, and responsibilities for data ownership, usage, and quality standards.
- Training: Education for employees on data quality principles and best practices.
Step 5: Demonstrate a Clear ROI
Calculate the projected return on investment. Consider these factors:
- Implementation costs: One-time technology purchases, setup fees, potential consulting costs.
- Ongoing costs: Software subscriptions, maintenance, and employee time dedicated to data quality.
- Projected benefits: Use your quantified costs of bad data and projected benefits of improvement.
- Timeframe: Estimate when the project can break even and start generating a positive ROI.
Conclusion
Data Quality as a Strategic Investment
Framing data quality as a strategic business investment is essential for securing the funding you need. By focusing on the language of business – increased revenue, cost reduction, customer satisfaction, and risk mitigation – you'll build a compelling case that resonates with decision-makers.
Taking Action to Secure Your Data Quality Budget
Don't wait for a major data-related disaster to strike. Start building your business case today. The sooner you invest in data quality, the sooner your organization will reap the benefits of better decisions, improved efficiency, and a stronger competitive edge.
Agile Data Warehouse Development
with automated test cases
Uncover the success story of Ifolor AG, a company that used BiG EVAL to validate data and gain trust in its decision-making process. See how data quality assurance can lead to a good gut feeling and get inspired by this compelling reference story today!
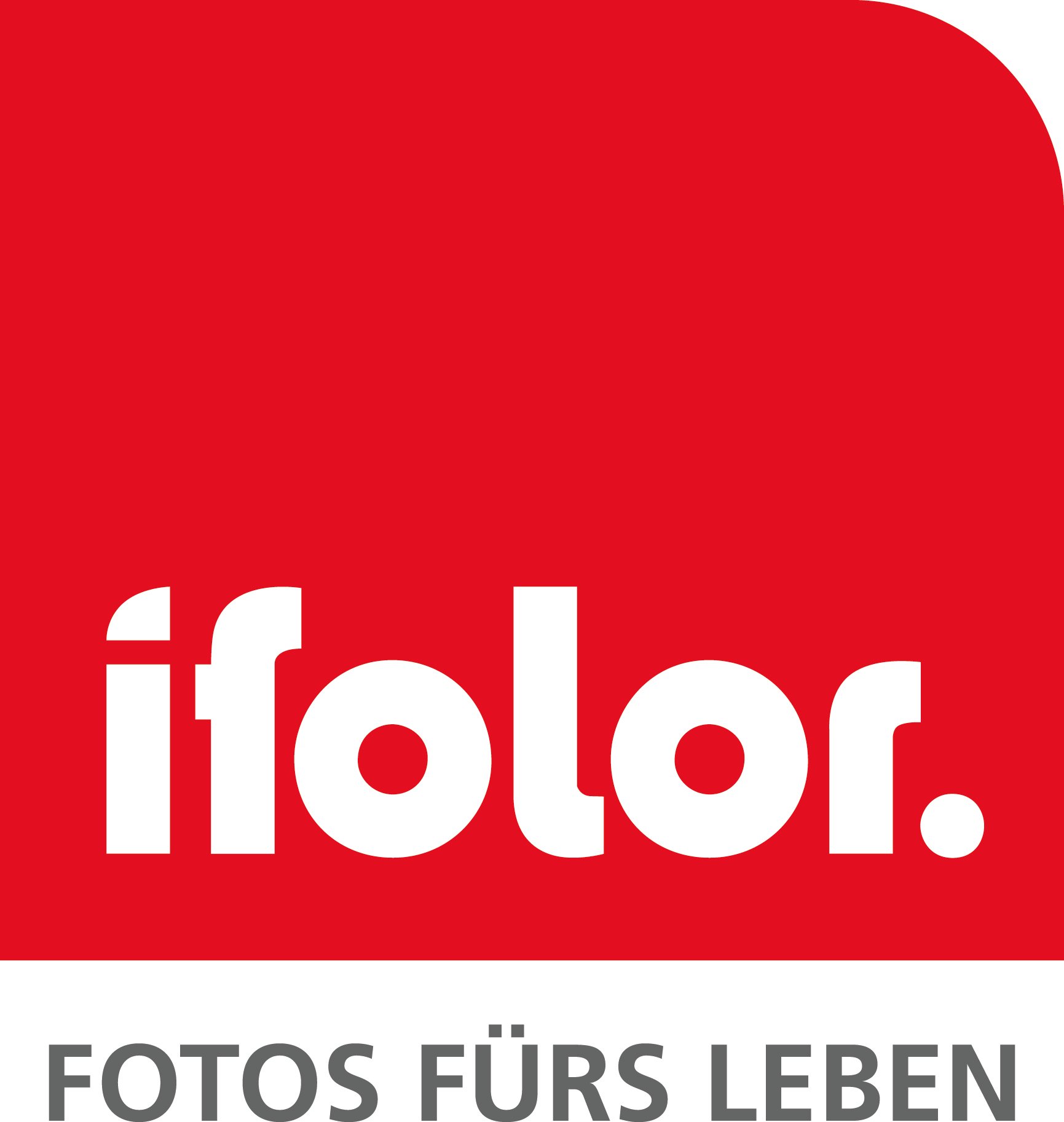
Get started today
Spend more time with your team pursuing big ideas and creating
valuable data solutions for your company.