Build or Buy a Data Validation Testing Solution?
Make the Right Decision
The perennial dilemma of "build vs. buy" applies to data quality and data validation testing as well. Is it better to take the time to build a custom solution in-house or buy a commercial alternative instead? Read until the end to learn our tips on which path to take.
What Preparatory Considerations Do You Need to Make?
Before you start building a data quality testing platform internally or procuring a commercial product, let us consider the preparation required. If you skip these steps, you will end up with an incomplete set of criteria to make your decision.
We will use the example of an organization looking to assure the quality of its business intelligence data, as this is one of the most common use cases we see in our practice.
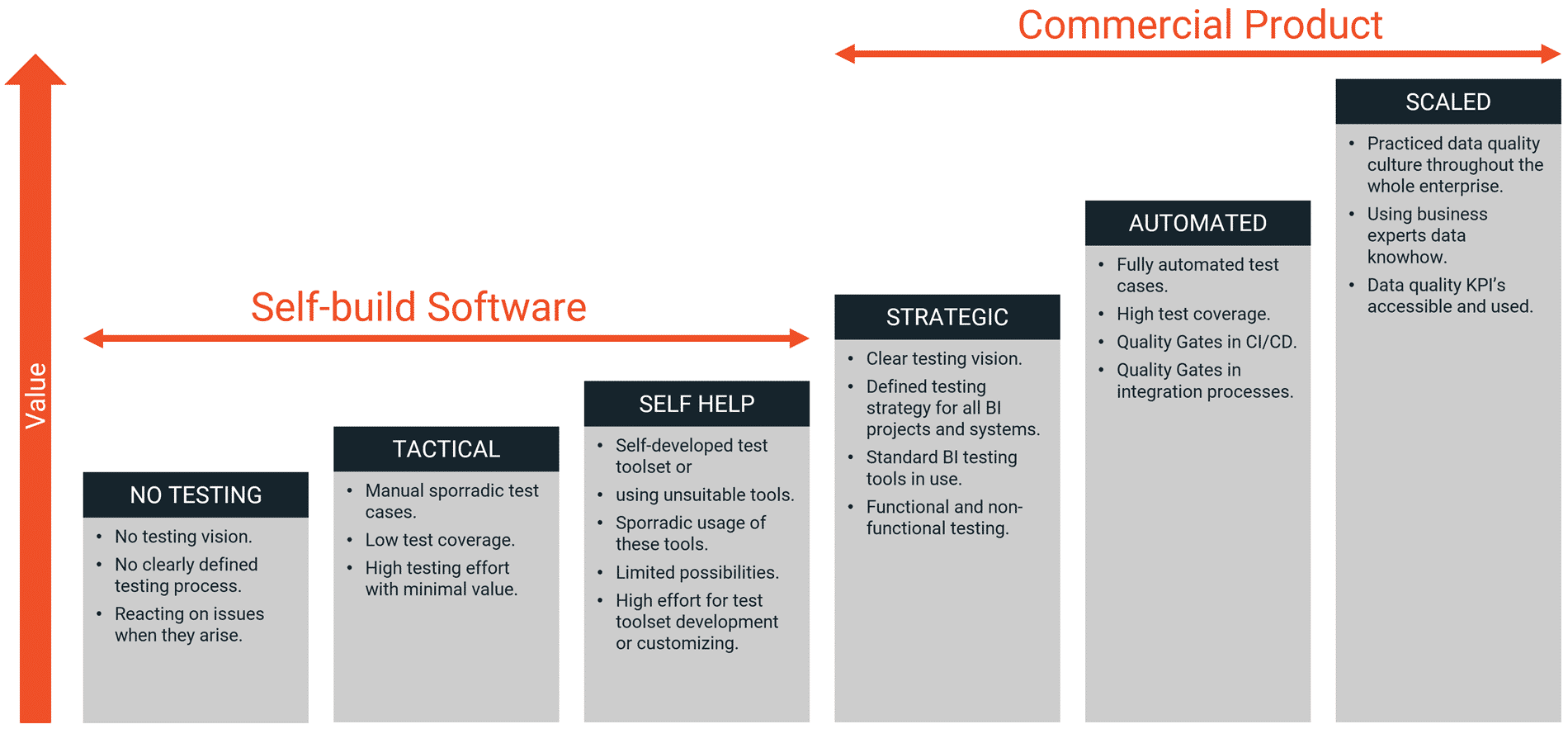
Consideration #1: What Are the Known Data Quality Issues?
You are likely considering a data quality testing solution because you have concerns about data integrity. Therefore, your first step is to catalog any known data quality issues. That should give you a better idea of what solution may resolve them.
For example, a past client reached out to us after senior management had become frustrated at ongoing discrepancies between financial reports produced within their business intelligence platform.
Poor data quality had eroded trust in their financial reporting system, causing managers and business intelligence architect team members to revert to Excel, undoing the investment and confidence placed in the new architecture.
Consideration #2: What Are the Consequences of Failing to Assure Data Quality?
As organizations swap Excel and manual reporting for more sophisticated, automated data analytics solutions, the speed at which errors can proliferate dramatically increases.
You need to consider the likely impact of poor data quality based on the issues you have already witnessed and the possible outcomes of future problems.
Many managers assume that their data is somehow assured and governed because they have a sophisticated data analytics platform.
Sadly, this is not the case.
Modern data platforms have thousands of processes and hundreds of system interfaces, any one of which could produce an error that poses significant risks to the organization.
It is vital that you fully understand the impact of poor quality data, both now and in the future. In doing so, you will clarify the ROI of implementing a robust data quality assurance capability.
Consideration #3: What Is a Reasonable Timeline for Putting a Data Assurance Capability in Place?
If data integrity or quality issues are apparent, there may be some urgency around building a data assurance capability. Therefore, it is worth clarifying how soon your stakeholders can expect a solution to be in place.
The reality is that in-house software development projects take significantly longer to implement. You may want to keep that in mind if urgency is required.
Consideration #4: What Data Solutions Have Been Created Internally Before? What Was the Outcome?
Building a data assurance platform is far more complex than a typical software development project.
Your organization may have an in-house IT team capable of building applications and simple data processes, but managing data quality across diverse data landscapes presents significant technical barriers that most IT teams have never encountered.
Note: You will learn what some of these challenges are later in the article.
If your organization has a history of creating complex data assurance and monitoring solutions, it is worth examining them to picture the resources and costs consumed accurately. You also want to clarify how effective these projects were at delivering against expectations.
However, if your organization has not developed similar data solutions in the past, it is unlikely that your IT team has the advanced data and software skills required.
Consideration #5: What Is the Current and Future Scope of Assurance?
Approximately 40% of the organizations that inquire about our data quality and assurance solution have considered building one in-house.
Many have even gone down the DIY path and only sought a commercial alternative after experiencing a negative outcome with their internally developed software.
One common difficulty with homegrown solutions is scope creep. The internal software appears to cope reasonably well within a minimal scope of simple data feeds and basic quality checks. But as demand for further assessment and monitoring grows, cracks soon appear.
It is important to realize that any solution needs to scale with forecasted demand. You can expect substantially more data feeds (both inbound and outbound), not to mention hundreds or even thousands of additional reports over time if assuring a business intelligence platform.
You can also expect other departments and use cases to emerge outside traditional data quality and testing requirements. Such use cases can include internal audits, regulatory reporting, user acceptance testing, and many more.
Ultimately, internally developed software will almost always lack the scalability of a modern, well-built assurance tool. Be realistic about the future growth and demand for data quality assurance and automated testing.
Understanding the Phases of Business Intelligence Testing Maturity
For an enterprise looking to test and assure its business intelligence, the next step is to understand where your organization sits on the maturity curve of testing and quality assurance.
Using the image below, you can gauge your current maturity level.
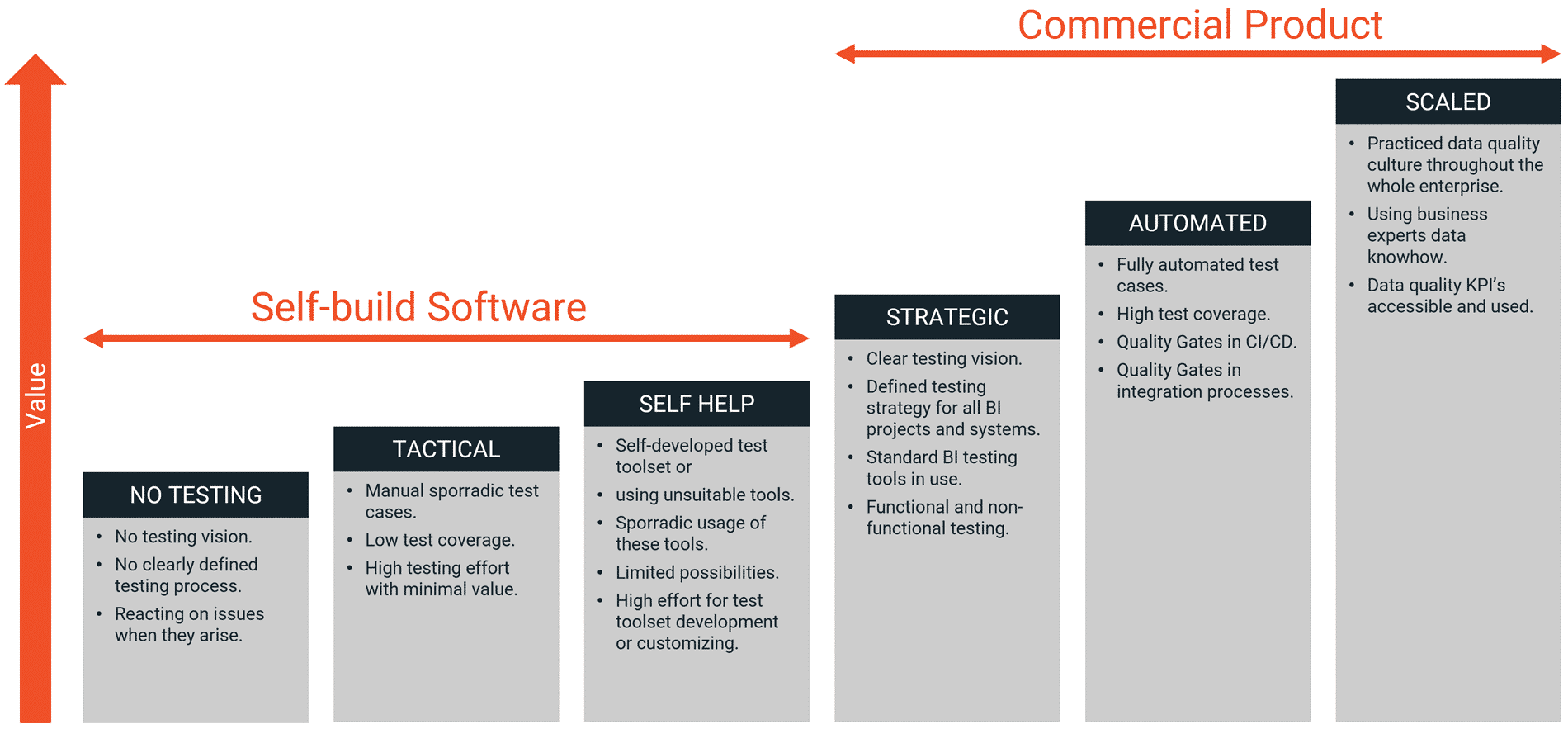
In our practice, most organizations that implement self-help solutions tend to have a weaker overall strategy for data quality and testing automation, which significantly increases the costs and risks to the business.
In contrast, companies that invest in appropriate technology are more likely to have a robust long-term data automation testing and quality assurance strategy, significantly increasing their ROI.
We also find that organizations that successfully scale their data testing and quality capability nearly always adopt a commercial solution as the foundation for the expansion.
Build vs. Buy: Essential Factors When Choosing a Data Validation Testing Solution
Now that the preparation is understood let us look into the merits of buying versus building data testing automation and quality assurance solutions.
The Cost Equation
One of the most compelling drivers for building a homegrown solution is the perceived lack of budget for buying a product off the shelf.
There is a misconception that developing an in-house solution can help avoid the upfront capital outlay of a commercial tool.
Sometimes, there are budgets allocated for internal IT development but not external capital investment. If you have an IT team of developers waiting for coding work, why not get them busy building a solution? When your organization has a freeze on capital procurement, developing an internal product could be your only path to a resolution.
However, solution providers like BiG EVAL significantly reduce capital outlay by offering a leased model. Our research shows that the annual cost of renting a commercial solution is lower than that of developing, testing, supporting, and maintaining one in-house.
For example, one organization had hired a developer/consultant to build a data automation testing framework and software solution. Several years later, they reached out for help. Working with their team, we replaced the legacy solution in less than 12 weeks and at a fraction of the cost spent on their previous approach.
Additional Concerns for Internally Built Software
When building an internal solution, you should not just consider the initial project cost. There are longer-term maintenance and support budgets that often get overlooked.
Internally built software often lacks standardization, making it more difficult to deploy across different use cases, technologies, and business units.
In contrast, commercial solutions like BiG EVAL can be exploited across a range of technical and business-focused initiatives. This "shareability" factor of the latest commercial software also means that funding and investment can be spread across department budgets, reducing the impact on one team.
In addition, we have found that commercial solutions provide more predictability around future spending. With internally created software, costs are difficult to predict because it is unclear how maintenance and development costs will rise as the scope and demand for data assurance increases.
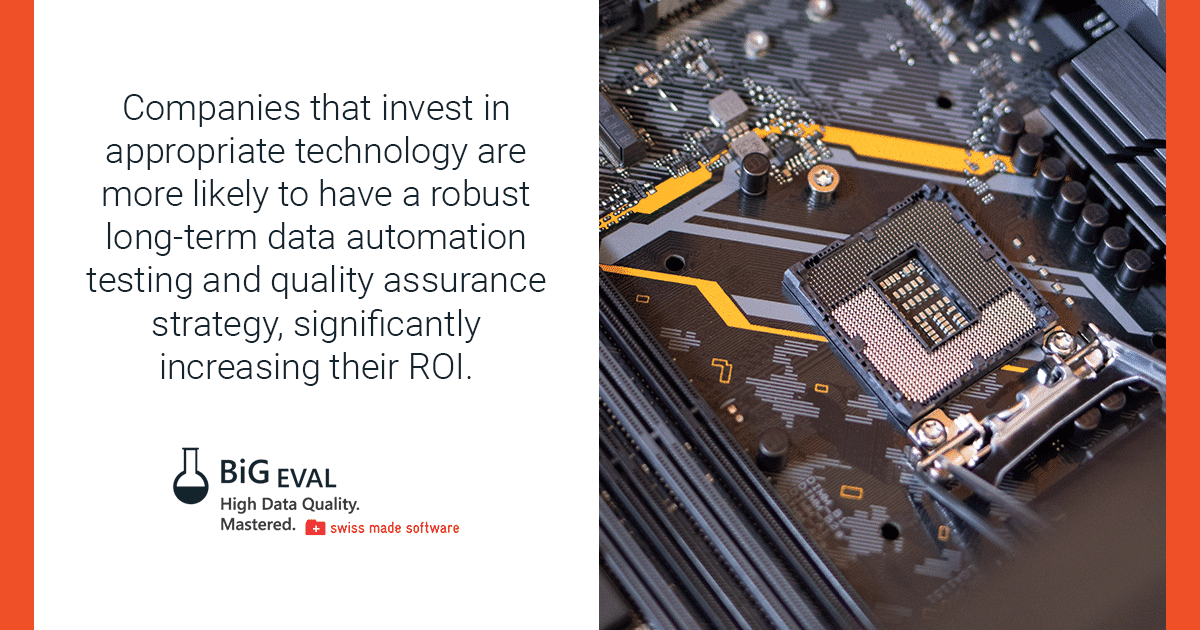
Productivity and Assurance Throughput
Perhaps the most apparent difference between "buy" and "build" is the same-day productivity of a solution like BiG EVAL.
This stark difference was apparent with a recent client who had previously carried out all their data assurance via SQL (a database querying language) and custom internal software designed to schedule and execute scripts.
Whenever a report or dashboard was requested using a new data source, a new data assurance process would take our client many days. The end-to-end process resulted in numerous handoffs between technical and business teams before deployment into a production environment. From there, even the most minor update would require several days of turnaround from internal IT, resulting in further productivity bottlenecks.
In contrast, using commercial data quality software such as BiG EVAL would enable them to implement new assurance processes on the same day they were requested.
This same-day productivity results in obvious cost savings for the IT team, but the real benefit is the productivity gain for the business. Each time your data platform is monitored for data quality, you target issues that will damage the business if ignored. Commercial data assurance tools increase your IT team's productivity and transform the performance of your data analytics and business services teams.
With a commercial solution, you can deploy data assurance processes in a fraction of the time required by custom-built internal solutions.
Functionality Portfolio
Clients often ask us to help them mature their data testing and assurance processes after building a homegrown software solution. In practically every case, we find that the software they have created has reached a dead-end in terms of its agility to cope with the modern demands of data assurance and quality testing.
It is easy to think that data assurance involves simple data checks to see if a value has been entered correctly or a process has been completed per requirement. If all you need are some basic validations, homegrown validation solutions may suffice for a tightly defined scope with a simple data source and processing chain.
However, the reality is quite different for most organizations.
At BiG EVAL, we have many years of experience in data warehouse and business intelligence data assurance testing. The diagram below highlights the most common use cases we encounter. The seven capabilities also indicate the functionality portfolio that we provide with our solution.
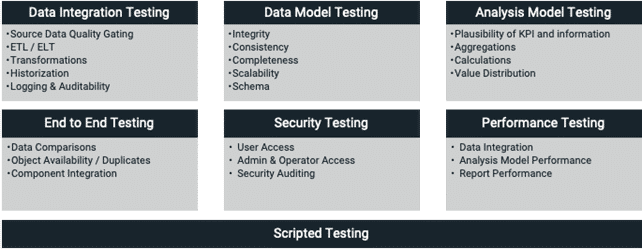
This image demonstrates that data testing has evolved substantially over the years. The modern data landscape is complex, varied, and constantly changing.
When you take on the burden of developing data testing and quality assurance internally, you commit to tackling the present data landscape and building a roadmap for whatever the future holds. Rarely is the average IT team equipped to deal with the full scope of functionality required, and sustaining that roadmap places significant pressure on already limited resources.Final Words
Perhaps the most compelling argument for building your own software comes from the point of supply and demand.
If your business and technical teams demand functionalities that commercial providers cannot supply, it makes sense to build your own solution.
However, supply is no longer an issue.
Modern, full-featured technologies like BiG EVAL address all the typical use cases for assuring and testing data.
Whether you require an end-to-end business intelligence data assurance solution or another form of in-flight data quality assessment, modern commercial solutions cost less than in-house development, deliver superior results, and provide far greater productivity.
Looking to Compare the Cost of Building Your Own Data Testing Solution Against a Commercial Alternative?
We will help you calculate our solution's total cost of ownership compared to the development and support costs of a traditional in-house build.
FREE eBook
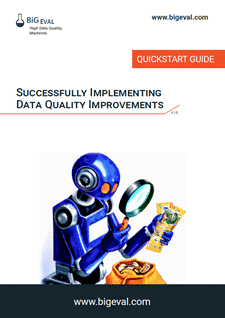
Successfully Implementing
Data Quality Improvements
This free eBook unveils one of the most important secrets of successful data quality improvement projects.
Do the first step! Get in touch with BiG EVAL...
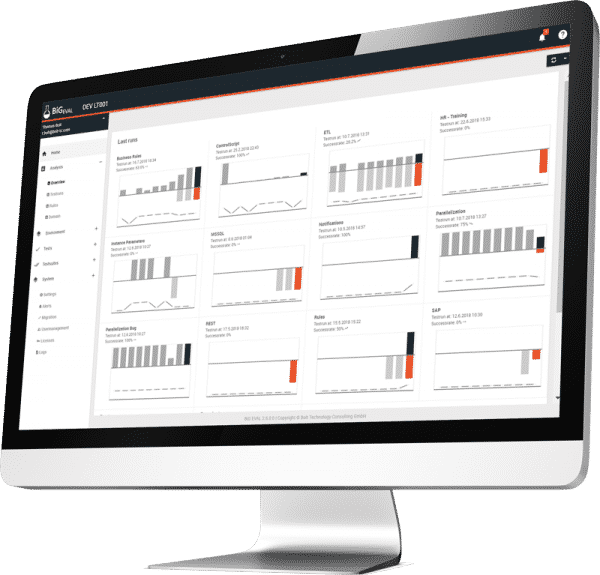
BLOG