Data Validation Testing: What Is It and Why Does It Really Matter?
Discover the essentials of data validation testing in this concise guide. Explore the different types and their importance in today's data-driven business landscape. Learn how to select and apply the right tests for accurate and reliable data, ensuring effective decision-making and error prevention. Ideal for professionals navigating the complexities of large data volumes, this article offers key insights into optimizing your data validation strategy.
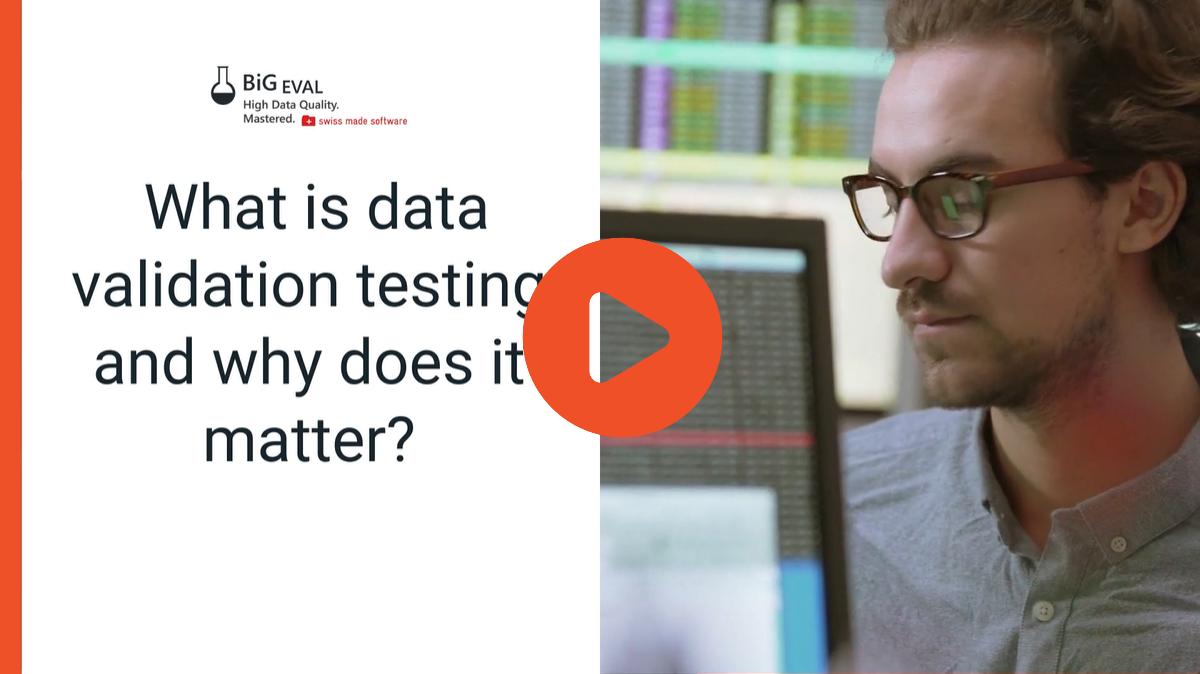
What Is Data Validation?
Data validation checks the accuracy and reliability of data before use, import, or processing. It ensures the data is clean, accurate, and relevant for informed decisions and achieving business goals.
There are various types of data validation, such as:
- Data integrity testing
- Data uniqueness testing
- Data consistency testing
- Data migration testing
- etc.
The appropriate type of data validation is decided based on the requirements, destination constraints, and/or data collection objectives. In today's fast-paced business world, companies demand access to big data to gain a competitive edge, assuming that the data is accurate and correctly interpreted.
However, the volume of data increases every second, making it harder and harder to manage. To balance out the complexities of ever-growing data, new techniques, business rules, and intelligence are used to enhance existing systems. This process is demanding, tedious, and error-prone. Data validation can help ensure that there are no errors along the way.
Why Data Validation Matters
When merging data from various sources, all repositories must be compatible and follow the same rules without corrupting data fields. Yet, inconsistencies in both the type and context of the data are common.
This is where data validation testing comes in. Its main goal is to ensure that merged data is accurate, consistent, complete, and free of data loss.
What Is Data Validation Testing?
The process of performing data validation as part of testing is called data validation testing.
The testing is performed on databases after applying transformations to them. That allows an end-user such as a business intelligence architect to check whether the available data is valid and databases are compatible and follow business rules and requirements.
Data validation testing makes sure that data integrity and data quality is not affected when extracting, transforming, and loading data. It also instructs end-users what to do with incorrect and inconsistent data in the form of test cases.
FREE eBook
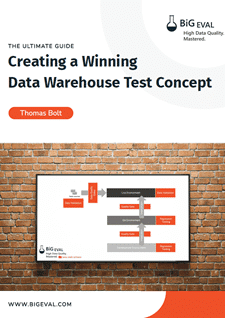
You want a Simple and Reliable Data Test Concept?
Download our latest ebook, "Creating a Winning Data Test Concept" and learn how to create a simple yet effective testing strategy.
Data Validation Testing for Enterprises and Data Integration (ETL) Projects
Big enterprises have to tackle huge amounts of data, and they must perform validation testing during the data collection process to make sure that it is not corrupted or compromised in any way before loading it into their data warehouse. Doing so helps guarantee the accuracy and integrity of the collected data, making sure that it can be used with confidence and no bad data is involved. It is also important to do data validation testing whenever data integration processes (ETL for example) are involved.
ETL (extract, transform, load) projects involve transferring data from one location to another, applying logical rules as needed and transforming it for use in the target location - usually a data warehouse. Validation testing is an important part of this process, as it helps to ensure that the data is accurate and free from any errors or discrepancies. It can also help monitor for any potential data losses and maintain consistency throughout the data pipeline.
Data Validation Testing for Migration Projects (Data Migration Testing)
Data validation testing is an essential step in a data migration project for business applications or data anyltics systems like a data warehouse for example. It helps ensure that the data being migrated from the old system to the new one is accurate, and consistent. As well as it ensures data completeness.
By conducting data validation testing during the migration process, potential errors and inconsistencies can be identified and addressed before they cause any major issues. This can help prevent data loss and ensure a smooth transition to the new system.
Additionally, data validation testing also helps identify any data quality issues, such as missing or duplicate records, that may need to be addressed before the migration.
Overall, data validation testing is crucial for the success of a data migration project and ensures that the migrated data is accurate and ready for use in the new system.
Common data validation tests
Verification testing
Also known as data comparison testing, compares pre-migrated and post-migrated data to check for any inconsistencies or discrepancies. This step ensures that data is accurately and completely transferred to the new system and that no data is lost or corrupted during migration. It helps identify and resolve errors in the migration process to ensure data integrity and reliability.
Domain validation testing
Business rule validation testing is a type of data validation that involves testing data against the business model and process logic of an organization. This type of testing is used to verify that the data adheres to the rules and constraints set by the business.
Range checks
This validates numeric values to ensure they stay within set ranges or limits.
Syntax checking
It helps to ensure that all records are correctly formatted and adhere to the necessary syntax guidelines. By verifying that the data follows the correct conventions, it allows for more accurate analysis and manipulation of the data.
Null checking
Ensuring there are no empty fields or missing information in a row of data records.
Checking the number of rows migrated
In a data-centric development project, data comparison and reconciliation involves comparing the number of records in the source and target systems to ensure they match and identifying any discrepancies that need to be addressed.
Functionality tests
Functional testing in data validation is checking that data meets the requirements and follows the business processes and logic. It ensures the data is valid and complete before it's used in any systems or processes.
Performance testing
It's the process of testing the speed, scalability, and stability of data systems and processes. It ensures the system can handle expected load and usage, preventing issues such as data loss, system crashes, and slow performance.
Security testing
Security is to ensure that data is protected from unauthorized access and breaches. This includes testing for data encryption, access controls, and vulnerabilities in systems handling sensitive data. It's essential to guarantee that the data is safe and confidential during the whole process from integration to analysis.
End to End validation
End-to-end testing in data validation involves testing the entire process from start to finish. It's essential in complex data-centric systems to ensure all data flows correctly, is transformed correctly, and is accessible by all relevant systems and users. This type of testing provides a comprehensive view of the data's journey and helps identify any issues that may arise during the process.
Regression tests
Regression testing in data analytics projects is a method of verifying that new changes or updates to the system do not negatively impact the existing functionality. This is important in agile projects where changes happen frequently, and it helps to ensure that the data continues to be reliable and accurate. Regression testing allows teams to catch and fix any issues early on, preventing them from becoming bigger problems down the line.
Data Validation Testing Steps
Data validation testing is an important part of any migration project. It helps to ensure that the data is accurate and free from any errors or discrepancies. The process consists of four main stages:
Detailed Planning
Detailed planning is an essential step in the data validation testing process. It involves creating a plan for the tests, so that all the data elements involved are accounted for and the most appropriate kinds of tests are used to validate them. When creating a plan, you need to consider which data elements need to be tested, what types of tests should be used, how much sample data needs to be tested, and how long the entire testing process will take. This is an important stage as it helps ensure that all issues are identified before proceeding with more comprehensive tests.
Database Validation
It's about verifying records, field types, and relationships between tables, protecting against incorrect decisions being made. It also guarantees that new entries are consistent with existing ones and no mismatches exist between related records. The comprehensive nature of this process enables early detection of errors to prevent major issues.
Data Formatting Validation
Data formatting validation is a crucial step to guarantee data integrity and accuracy. It verifies that all data fields in database records are consistent with their expected formats, eliminating any potential for manipulation. By keeping information consistent across entries, it helps reduce errors during the query stage and avoids conflicts between related records. Data formatting validation is an essential part of the data validation process.
Sampling
Data sampling is a valuable tool for validating data. By running samples of a dataset, it enables users to check for any potential problems before conducting more comprehensive tests on the entire set. This helps to improve accuracy and reliability when analyzing and manipulating records, as any issues uncovered at this stage can be promptly addressed. Additionally, sampling enables users to quickly test out new algorithms or models on smaller datasets without having to process their entire dataset at once.
Benefits of Data Validation Testing
The benefits of data validation and related testing approaches for most companies are numerous.
- Ensures data accuracy and completeness
- Detects and prevents bad data
- Improves data quality
- Enhances data consistency
- Increases data reliability
- Enhances data security
- Optimizes data performance
- Enhances data integrity
- Improves data analysis and reporting
- Enhances compliance with industry regulations
- Increases efficiency and cost savings
- Reduces the risk of data breaches and security issues.
What Is Database Validation Testing?
Other than data validation, database validation is also important. Database validation testing involves stored data and metadata validation. The testing is done based on requirements against the quality and performance of the data. Testers also look into the data objects, functionality, types, and lengths before making the data live and available for users. Indexes and the entire environment where data will be moving and evolving are also checked against set parameters.
Common types of database validation testing include:
- Data mapping
- ACID validation
- Data integrity checks
- Business rule compliance tests
- Data accuracy tests
- Data completeness tests
- Data transformation tests
- Data quality tests
- Database comparison test (comparison between source and target)
- End-to-end tests
- Data warehouse tests
These types of proactive and continuous testing can help prevent data errors.
FREE eBook
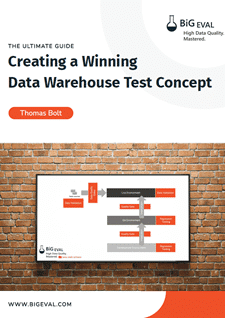
You want a Simple and Reliable Data Test Concept?
Download our latest ebook, "Creating a Winning Data Test Concept" and learn how to create a simple yet effective testing strategy.
Steps to Adopt Data Validation Testing
Data validation testing is a critical step in ensuring the quality and accuracy of your data. But with so many different tests to consider, it can be difficult to know where to start. Fortunately, there are a few key tests that can easily be incorporated into your workflow to help streamline the process.
For example, data accuracy and completeness tests ensure that the data is correct and complete, while data transformation tests verify that the data is not corrupted during complex data mapping. Data quality tests then help to identify and handle any bad data that may have slipped through.
Additionally, database comparison tests allow you to compare the source and target database, while end-to-end and data warehouse tests help with data validation in more complex data transformation scenarios.
But don't let the number of steps involved discourage you. Incorporating data validation testing into your workflow is a must for companies handling big and complex data. With the right tools, like BiG EVAL, data validation can be effortless and efficient. The end goal is to guarantee correct business intelligence and optimal return on investment.
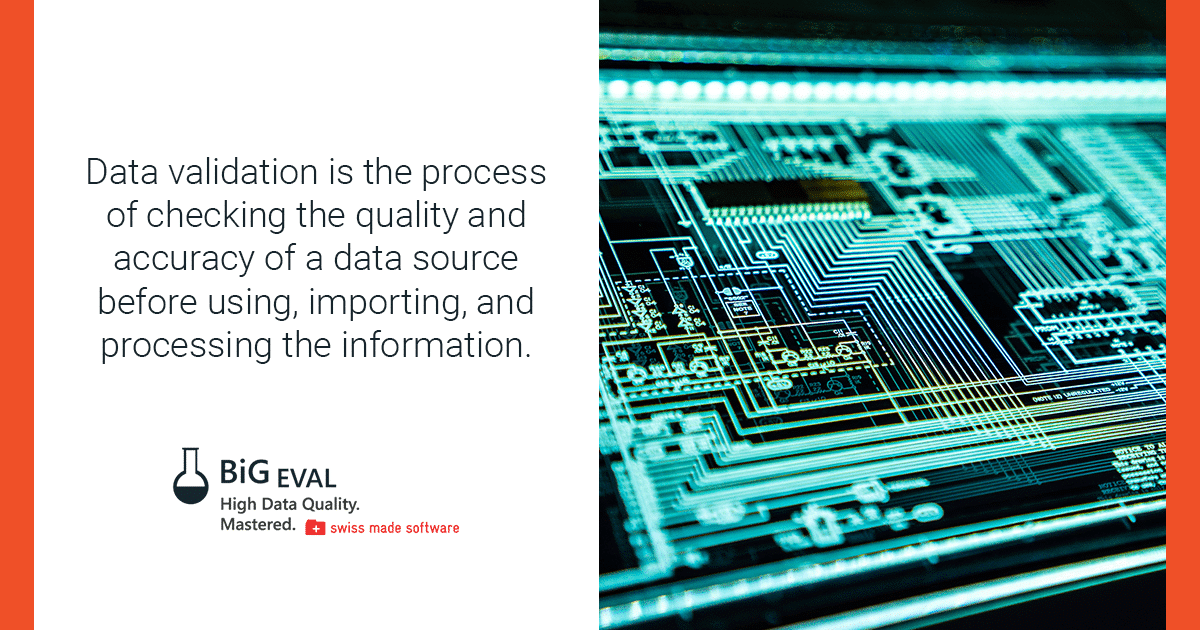
What Kind of Software Is Needed to Validate Data and Ensure Data Integrity?
It really depends on your requirements. You may start with more manual testing tasks that you may support with Excel and maybe Power Query. But however, these processes still require considerable thought, processing, and effort to shape the data.
Because efficient, accurate data management is so vital for business intelligence, specialized software has been developed to help meet enterprise data needs.
BiG EVAL is one of these tools that support data validation testing in an optimal way. It automates test processes in data-centric projects like data migrations, data warehouses, imports, exports and many more. Best Practices templates collected from hundreds of BiG EVAL's customers who have done data validation testing before, make the process even easier and more efficient for you.