Learn common ETL data quality issues and solutions to improve data integrity and analytics reliability.
Data Testautomation
These strategies and tools help you optimizing the development-quality within data oriented projects.
High valued results with data-analysis and data-processing can only exist when the system-components are error-free. Ensuring a high quality when developing these components can be time-consuming and needs a strong testing strategy. Automation tools that leverage the efficiency and free your developers from testing tasks are essential as well.
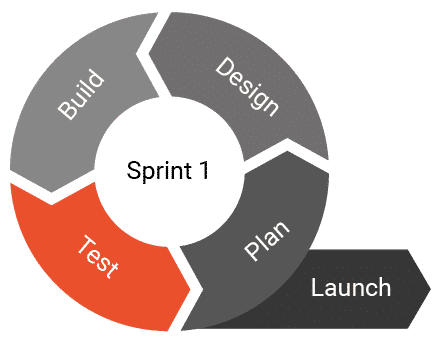
Software-Solution
BiG EVAL Data Test Automation
The software solution BiG EVAL DTA automates testprocesses in data oriented projects like data warehouses with ETL or ELT processes, data migrations, data imports and exports, data integration interfaces and many more. No matter how many test cases are made!
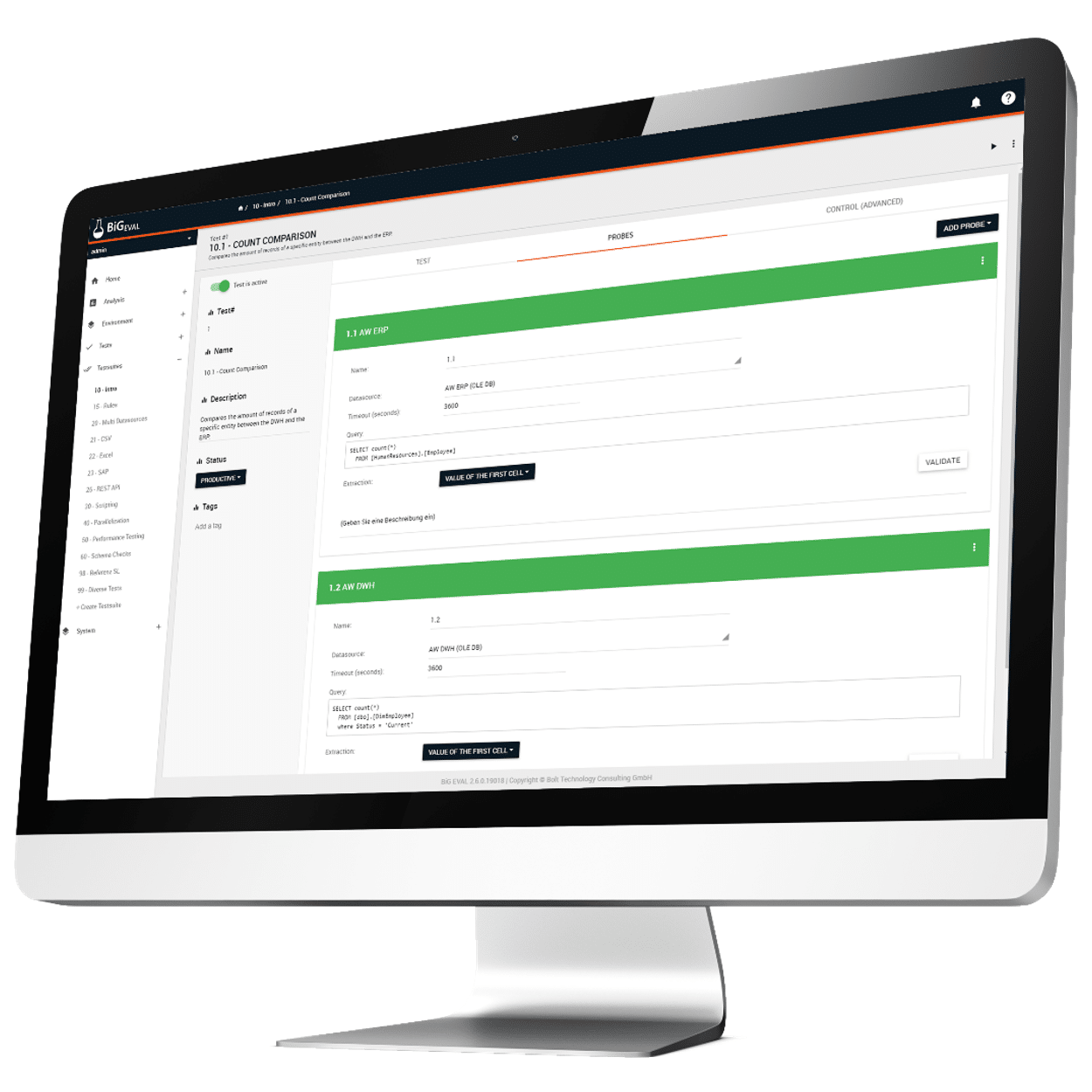
FREE Exclusive DQA Club
Discover the Secrets of Data Quality Automation
Get to learn every secret of Data Testing and Data Quality Management directly from our experts and partners that are working in the field.
Apply to be a member of our DQA club today and join the circle of competent specialists.
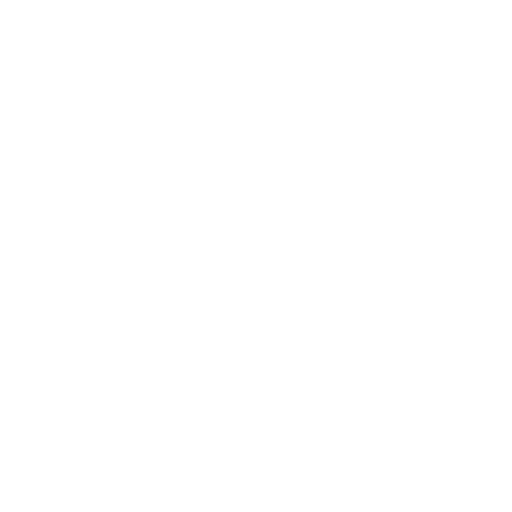
Expert Articles
Here you find the best articles our experts published, that support and consult you in defining and implementing your data test strategy.
Mastering Test Automation: A Game-Changer for Software Quality and Efficiency
Explore this guide to test automation, covering key concepts, tools, and best practices. Master the art of automating tests for reliable software delivery.
[...]Data Product Testing Bottlenecks: How to Achieve Massive Scaling the Easy Way
Discover how to streamline data product testing, achieve max coverage, and improve quality through the power of massive scaling.
[...]Costs for Quality Assurance and Testing in Data Product Projects
Bad data costs big money. Learn how Quality Assurance and Testing in your Data Product Projects saves you cash.
[...]How BiG EVAL Supports Continuous Integration and Delivery in Data-Centric Projects
BiG EVAL’s automated testing helps support continuous integration/delivery CI/CD processes, ensuring quality assurance during deployment.
[...]How Your Business Will Benefit from Moving Your Data Warehouse to the Cloud
What are the advantages of migrating a data warehouse to the cloud? Learn how automated data validation tools ensure the quality of your migration.
[...]Is a SQL Minus Query helpful for efficient data validation?
Learn about SQL Minus Queries in data validation. Are they efficient? Are they reliable? Do they make sense in your specific scenario?
[...]Power up End-to-End Data Validation with a Technology-Agnostic solution
End-to-end testing for data analytics components with a technology-agnostic tool is crucial to provide the most benefits to business.
[...]A Fresh Look: Do Developers Need Outside Data Testing Automation?
A Fresh Look: Do Developers Need Outside Data Testing Automation?Everyone can agree that data testing is essential to ensure that all the parts of your data-oriented product will work properly throughout its lifetime. But is it better to outsource data testing or keep it in-house? Everyone can agree that data testing is essential to ensure […]
Why Data Warehouse Testing Automation should start in the Staging Area
Why Data Warehouse Testing Automation Should Start in the Staging AreaStarting to data test within the staging area, right where data enters your data warehouse integration process, is essential. Data staging is an essential step in DataOps and data warehouse testing automation specifically. Staging areas provide dedicated spaces to safely test, transform, and cleanse data […]
How data warehouse testing helps prevent sudden load process breaks
How data warehouse testing helps prevent sudden load process breaksSuccessfully loading data from source systems into a data warehouse heavily depends on the structure (meta data) of the source database or files. It is a kind of interface for a data integration or ETL process. And as such, it is an external dependency, the data […]
Data Warehouse Test Automation 101: What Are Data Integration Loads?
Data Warehouse Test Automation 101: What Are Data Integration Loads?Data loading is a complicated and delicate process with considerable potential for errors, inconsistencies, and anomalies. To maximize data quality, consider implementing data warehouse test automation. What Is Data Loading?Data loading is the process of copying and loading digital data from one or multiple sources, such […]
Data Catalog: What It Is and Why You Need One
Data Catalog: What It Is and Why You Need One According to a report by IDC, the global datasphere is predicted to grow from 33 zettabytes in 2018 to 175 Zettabytes in 2025. What this means is that companies now have access to enormous data spanning multiple departments and sources. However, companies still face two main challenges […]
Build or Buy a Data Validation Testing Solution? Make the Right Decision
Should you buy a data quality automation solution or build your own? This article discusses the pros and cons and shows what has to be considered.
[...]How to Create a DataOps Process for Data Testing with Data Quality Software
Take a look at DataOps and see how to operationalize Data Quality and Data Testing in a complex Data Warehouse environment.
[...]Data Validation: What, How, Why?
Data Validation: What, How, Why?Have you ever wanted a way to ensure the data you collect is accurate, qualitative, and healthy? If you have, you’re not alone. Fortunately, data validation testing already provides a solution to the problem. Studies show that many enterprises base their decisions on outdated, uncorroborated data, which has negatively impacted their […]
Advanced Analytics vs. Business Intelligence
Advanced Analytics vs. Business Intelligence Advanced Analytics and its ability to enhance revenues What is analysis?Complex systems need to be broken down into smaller modules to make more sense out of them. The analysis involved focusing on smaller modules to understand the intricacies.What is analytics?The study of data sets, facts, and figures to deduce meaningful patterns […]