Getting a competitive edge utilizing a high data quality
What is data quality and why is it important?
Benefits, tools and talk with experts.......
Using data as a foundation for strategic decisions and utilizing it in business processes, gives companies a competitive edge. The higher the quality of your data is, the higher the value for the business will be. To stand out between your competitors, one key factor is surely the quality of your data that drives your business.
What is Data Quality?
For organizations, measuring data quality is no longer an option. Mainly because of its ability to make the organization stand out from the competitors and be on the top of their game. It helps firms to identify and rectify data errors in the starting phases of the lifecycle, which eventually makes sure that their data is compatible with their IT systems to best fit their intended use and purpose. The need to assess data quality has also picked up the pace due to its linked advantages on business success as data-driven decision making makes a company more successful. Managers and decision-makers now highly rely on these models to make important business decisions.
Data Quality can be measured to assess the condition of the data in hand. Multiple factors contribute to determining the quality of data such as completeness, reliability, accuracy, and consistency. Other than these, data needs to be up to date and fresh.
Data Quality Management (DQM) is the main component of the entire data management process, and constant efforts to improve the data quality give the basic idea to Data Governance which makes sure the firm uses consistent and coherent data in all departments and levels.
Why is it important?
Data quality cannot be compromised due to a heavy reliance on data models to make business decisions. Poor data quality can not only lead to negative consequences and heavy financial losses, but it can also potentially tarnish the repetition of the entire organization due to weak business strategies and errors in marketing campaigns.
Imagine a supermarket deciding discount percentage for the year-end sales considering its yearly numbers. One single piece of wrong information can create a discount value that might not suit the business, eventually causing monetary loss. On the other hand, wrong data on an e-commerce website can make the customers go away and eventually tarnishing the entire business reputation.
A study by Thomas Redman in the MIT Sloan Management Review 2017 shows that 15 to 20% of annual revenue is spent on correcting errors caused by bad quality data.
The need to pay attention to data quality and its continuous improvement is due to its linked advantages on business success as data-driven organizations tend to make better decisions based on business intelligence and data analytics models. Managers and decision-makers now highly rely on these models to make important business decisions.
Here you can read about, how some well-known companies have sustainably improved their data quality:
What is good quality data?
Now the question of identifying good quality data arises. What means good? It has some attributes that prove its authenticity and integrity. A good quality data is integral if it is accurate and predicts accurate outcomes. It eliminates transactional processing issues in operational subsystems and thus provide accurate results in analytics-based applications and computations.
For this to happen successfully, incorrect data and low-quality data need to be identified and rectified. Documenting data issues drastically helps data analysts to get good quality data which ensures that officials are working with good information to get reliable business results.
Data Quality is also ensured by data completeness, data consistency, data currency, and absence of redundancy. These are the major attributes that a good quality data set has and that make it trustworthy and reliable.
Data Completeness: Data set containing all the recorded data.
Data Consistency: Absence of conflicts between values and redundancy.
Data Currency: Data set containing all the updated and current values in the correct format.
Characteristics to determine data quality(dq)
- Data quality can be assessed by looking at some distinct characteristics of the data set. Benchmarking of results generated by using bad or good quality data sets is one of the most reliable methods. These benchmarks provide a baseline for result comparisons and data quality issues in the new data sets.
- Another way to determine it, is by having organization-related business rules. For example, the Telecom industry has its set standards to determine dq of data sets and the Machine Learning software development industry has its standards. The data management teams usually conduct assessments to determine their desired data quality and keep all the processes and findings documented to avoid further errors. This practice can be done multiple times annually to keep the data in its highest degree possible.
- For example, UHG (United Health Group) performs Data Quality Assessment and have published the framework they use to measure it, for the benefit of other companies.
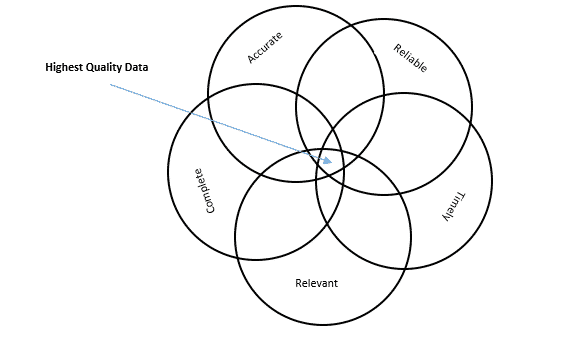
Let’s enlist and elaborate on the characteristics of good quality data. All topics represent a dq- measure that defines the correctness of the data set:
- Accuracy: An accurate piece of information is the one which is coherent with the real-world problem-solving data. It is a critical data quality dimension as it can cause major consequences if ignored.
- Completeness: A complete piece of information contains all the fields that are required to make sense of the data. It is an important data quality characteristic as incomplete data is unusable.
- Reliability:A reliable data does not contradict with other data sets. Lack of reliability results in untrustworthiness in the data and analysts cannot depend on such data.
- Relevance: It measure and defines the reason for the collection of the data set. It is important to collect only relevant good quality data, otherwise it is a waste of resources such as money, human efforts, and time.
- Timeliness: It measure and defines the up-to-datedness of the data set. It is an important data quality dimension as often outdated data creates problems, as well as, new data comes and old data become redundant.
Who are data analysts and what do they do?
The task of data analysts is to understand data and find useful patterns in it. This analysts study complex data and transform it into meaningful information. These are the building blocks of business insights that drive business decisions. Data quality analysts examine the performance of the models built around the data set. A close relationship needs to be developed with database engineers and developers to design and implement automation processes. A good data quality analyst loves to solve problems, has great analytical skills, can find patterns in the data sets, have an eye on new computer system researches, and who is a pro at mathematics.
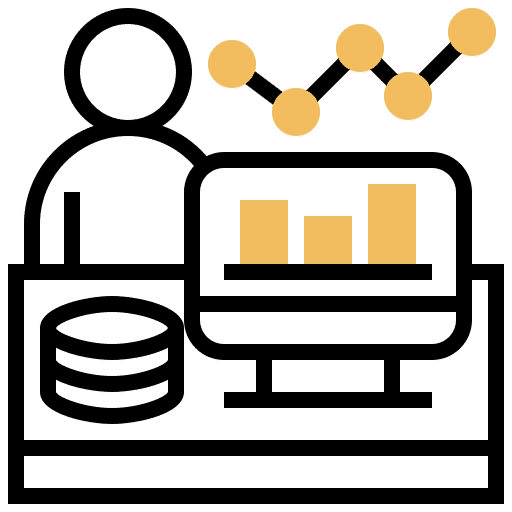
Depending on the industry of an organization, the role of data analysts may vary thus the exact job description and role are variable. For example, in an IT firm, data analysts successfully delivers; data analysis, business logic building, test plans, test scripts, requirement analysis, database logics, and more.
Based on common job listings on recruitment websites, it can be analyzed, that a DQ- analyst’s duties typically involve:
1. Interpretation of Data.
Statistical techniques help data quality analysts to interpret the data and observe the authenticity of the results so that upper management can take advantageous decisions.
2. Research & Development of the Database design.
For effective data collection, storage, and fetching, data quality has to be maintained which is the task of data quality analysts. They sit with the database administrators, designers, and developers so that data to implement effective strategies and optimizations are readily available for the firm and the best quality data is captured in the first place.
3. Identification of Trends.
Complex data sets have underlying trends and patterns in them that need to be identified by a person with great analytics and problem-solving skills. Thus this task is given to data quality analyst. Both primary and secondary sources of data are maintained to check the best data quality and maintain database systems.
Review of Reports.
Data needs to be reviewed and cleaned before it becomes usable. Clean data ensures better performance and better results. Reviewing results and reports to correct the data collection and cleaning phases is also a job of the data quality analysts.
4. Identifying business needs and prioritizing them.
To understand what is required from the data, data analysts need to sit down and prioritize the firm’s business needs. This ensures the required data is collected and processed.
Data quality management Software tools and techniques
There are numerous data quality management tools and techniques that help DQ- analysts perform their tasks efficiently. Firms treat the process of data quality management as projects with multiple steps. The steps in the dq- process are:
Data Quality Management Software tools have the power which humans don’t have. Data quality software measurement tools can replace human efforts and can easily match records, validate incoming data, delete duplicate data and perform identification of all kinds of data in the data sets. Data Profiling is also done easily by Data Quality Management Software tools. Profiling finds outlier values in the data.
Data Quality programs support; data handling rules creation, data relationship discovery and automation of data transformations. This is how automation helps in data quality maintenance.
There is another kind of tool called collaboration enablement tools. They have recently become more widely famous as they provide a common view of data repositories to the officials who are data quality managers. These Data Quality Management Software tools improve the quality maintenance lifecycle as well as improve the usability of the data sets. Often, companies use data governance programs that occasionally use metrics to assess the quality of the data and produce master data management (MDM) reports. This creates a centralized and standardized way to process data.
Benefits of good data quality
Maintaining good quality data foremost has some major financial benefits. Enterprises are reducing their error identification and rectification costs by using data-driven models to enhance their systems and making smart decisions. Enterprises are also avoiding overheads and operational costs due to the efficient running of their businesses by predicting breakpoints and faults.
Additionally, the accuracy of analytical applications gets improved which leads to better decisions in terms of business strategies. This increases the revenue and competitive edge of the company against its competitors. The Data Quality Management tool BiG EVAL, give better and trustworthy findings. in just one click!
With the help of effective data management due to good quality, management teams can focus their energies and efforts into monetory action items rather than cleaning up data sets. Productive alternative activities can be making strategic and analytically sound decisions, promotions, and maximization of yield.
Challenges of data quality management
Despite of all these benefits good data quality brings, why is it that many companies are not focusing on data quality? It is because maintaining the best data quality comes with some challenges. The structural and relational databases need to be defined with the long term vision of good quality data gathering in mind. The advent of Big Data also comes with its extracting, transforming and loading challenges. Data quality managers need to continuously focus on maintaining unstructured and structured data such as logs, sensor data streams, records, and texts.
BiG EVAL DQM support these processes and make them much more efficient.
On top of that, prediction models using machine learning algorithms are used in artificial intelligent systems. Feeding bad data to these models lead to wrong decisions or recommendations of the artificial intelligence. That`s where everything begins !
And much more. The models learn from these bad decisions. This kind of problem is completely new and was not present 5 years ago.
Organizations need to adopt real-time streaming platforms to cater to large volumes of complicated data. The advent of cloud based data sets has further increased data quality management complications.
Network security reasons also complicate data quality demands as data privacy and protection has to be ensured at each step.
All in all, maintaining good data quality is crucial for companies to maximize their productivity using data-driven models but it requires some effort by the DQ- analysts to eliminate the complications and acquire the countless added benefits that come with it.