Data Governance: A Comprehensive Guide
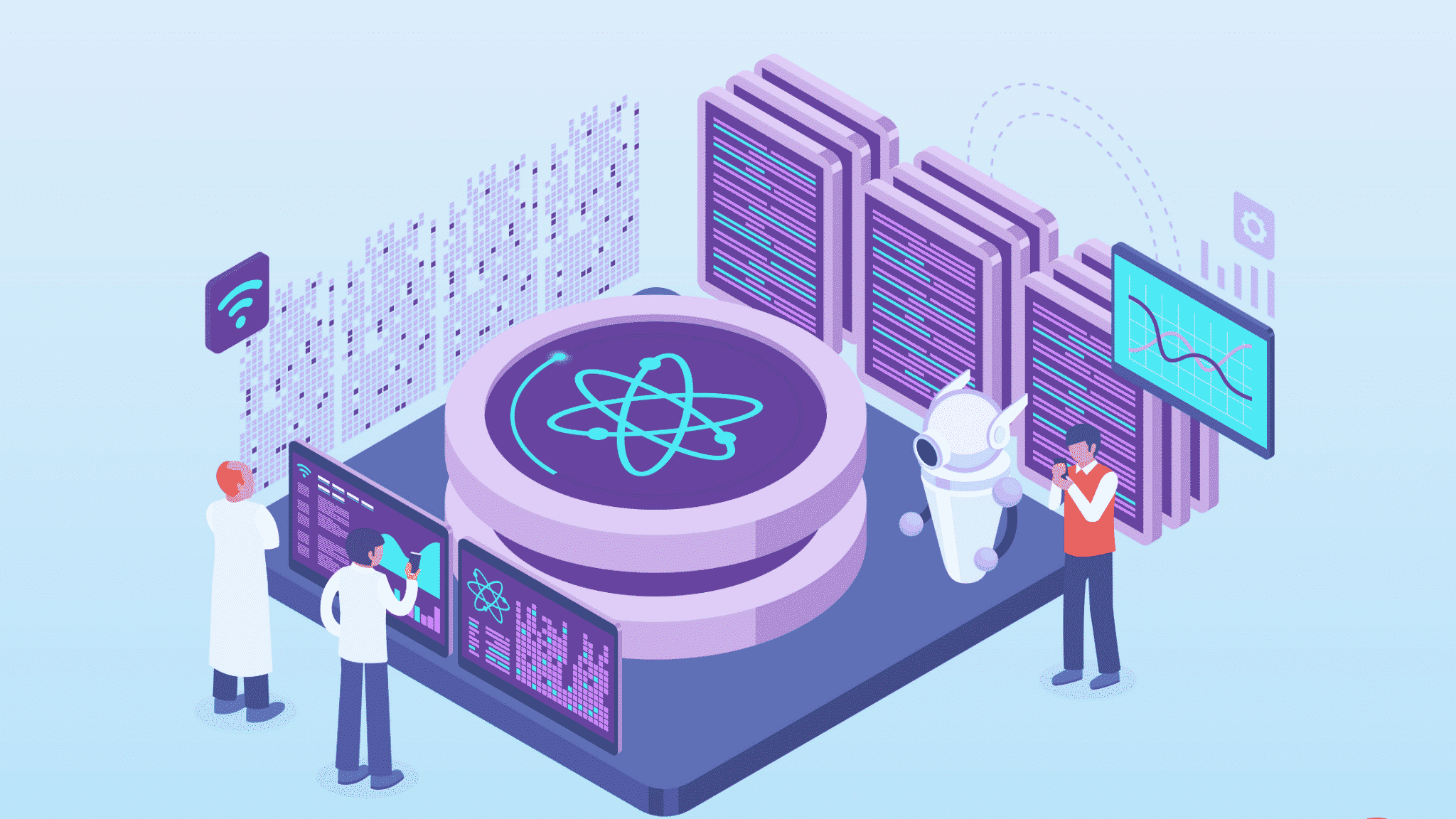
Enterprises need to plan for the data they create so that it is handled consistently, in a manner that supports business outcomes. The institutions that succeed at this ensure security and compliance and gain value from the information they collect and store.
Studies show that data governance is one of the leading strategic initiatives for multinational organizations. Since new technological externs like artificial intelligence and machine learning need high-quality data, it's unlikely this trend will change any time soon. In this article, you'll learn more about it.
Contents
What’s the difference between data governance and information governance?
Data governance benefits and goals
Data governance models (frameworks)
Is your organization ready for Data governance?
Data governance certifications
What is data governance?
Data governance is a process that facilitates the management of the availability, integrity, usability, and security of data in corporate systems, based on internal data policies and standards that extend to data usage. This data governance definition also applies to big data governance, the only difference being that big data governance applies only to large volumes of data.
Functional data governance ensures that data is trustworthy, consistent, and not used for the wrong purposes. It is increasingly vital as organizations are subjected to new data privacy regulations and depend more on data analytics to enhance operations and gather insights for business decisions.
A properly designed data governance program usually comprises of a governance team, a steering committee that functions as the governing body, and a data stewards’ group. They collaborate to develop the policies and standards for data governance, as well as the enforcement and implementation of procedures.
What’s the difference between data governance and information governance?
Information governance deals with the business and compliance-based approach to managing the usage, retention, and disposition of business records.
According to the Information Governance Initiative, information governance encompasses the “technologies and activities organizations implement to maximize the value of their information while minimizing the accompanying costs and risks.
Information governance covers both unstructured and structured data. It involves elements such as information lifecycle, categorization, the definitions of use, secure disposition, and information access, and is largely the responsibility of an organization’s management.
In contrast, Data governance is more of an IT responsibility. It applies mostly to data transfer and storage. The many facets of data governance include data lineage, data security, data service levels, data management, and data loss prevention.
To comprehend the differences between information governance and data governance, it helps to observe the skills required by each field. Information governance requires specialists with a thorough understanding of records and information management, technology, privacy, collaboration, discovery, and disposition. In contrast, data governance professionals should be proficient in data architecture, data privacy, data modeling, data management, and data integration.
Data governance benefits and goals.
The key aim of data governance is to eliminate data silos within an organization. Silos typically develop when individual business units implement separate transaction process systems without a centrally coordinated strategy or enterprise data architecture. And it seeks to streamline the data in those systems through a cooperative process, with stakeholders from each business unit taking part in the process.
Another goal of data governance is to ensure that data is utilized in the right way to prevent the introduction of data errors and prevent potential abuse of personal data about customers and other sensitive details. It is usually achieved by creating consistent policies on the usage of data and establishing procedures to evaluate usage and enforce the policies on a continuous basis.
Without adequate data control, data inconsistencies across the different information systems run by an organization might go unnoticed. For instance, customer names may be listed inconsistently in logistics, sales, and customer service systems. This may hinder any attempts at data integration and create data integrity problems that affect the reliability of business intelligence, analytics applications, and enterprise reporting.
Data governance failures may also hinder efforts to comply with regulatory changes, which could prove problematic for companies that need to adopt new data privacy and protection guidelines, like the European Union's GDPR and the California consumer privacy act.
An enterprise data governance initiative typically leads to the development of standard data formats and common definitions applicable to all business systems, consequently improving consistency for both business and compliance purposes.
Do the first step! Get in touch with BiG EVAL...
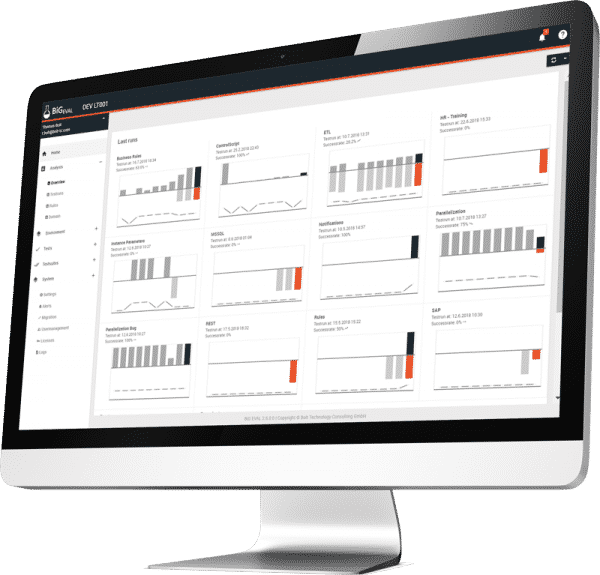
Data governance models (frameworks).
In essence, a data governance framework is a model for enterprise data management. The framework provides guidelines and terms of engagement for management and business activities, particularly those that require or result in the manipulation and creation of data.
Centralized governance (both single and multiple business unit scenarios)
Centralized governance is defined by single or multiple enterprise units that consolidate the maintenance of master data. In this framework, one central node handles setting up master data based on requests from data consumers. It is suitable for large-medium sized organizations with multiple facilities.
Benefits
- Master data has a longer lifespan
Disadvantages
- There are complex data requirements.
- It requires several legal permissions and must adhere to extrinsic factors like government law.
This data governance framework provides extensive control over master data. However, it often comes with delays and requires a large, formal data governance department. That said, the data created is highly consistent, and it is easier to introduce changes and process improvements since few users can set up master data.
Centralized data governance and decentralized execution
In this model, a centralized data governance authority defines the framework of controls, and individual business units create specific parts of the master data. This model is ideal for large-medium sized organizations with multiple facilities.
Benefits
- It gives master data a long lifespan.
- It permits master data sharing with other business units.
Disadvantages
- It requires multiple legal permissions and is affected by extrinsic factors like government regulations.
While this data governance model provides significant agility, organizations must establish proper controls where necessary.
Decentralized execution (single business unit)
This data governance strategy requires individual business users to manage their own master data. This model ensures that data is created by local users. It is ideal for small enterprises like a single company or manufacturing plant.
Benefits
- Data maintenance procedures are relatively simple.
Disadvantages
- Business units can’t share master data.
- The master data has a short lifespan than other data models.
Though this model is more straightforward and can be set up faster, there can be significant data inconsistencies if it is not managed correctly.
Here's how to avoid this problem.
- Ensure data ownership is clearly defined and that it is restricted to a handful of experts within the enterprise.
- Provide clear instructions for how each field should be populated, along with explanations on the meaning of each value for each field.
- If your budget allows, invest in automated tools to ensure data consistency.
- Establish controls and audits to quickly identify and correct data inconsistencies.
Decentralized execution (several business units)
In this model, multiple business units collaborate with shared materials, customers, and vendors. It is best suited to small and medium-sized organizations with several facilities.
Benefits
- Data maintenance tasks are relatively simple.
- It allows master data sharing with other business units.
Disadvantages
Master data has a short life span.
Note: this model can create major data inconsistencies since multiple parties are involved. This problem may manifest in the form of duplicate master data and may prove frustrating. To keep this from happening, you should;
- use automated tools that ensure data consistency - regardless of who the original creator is.
- Restrict the number of fields that require maintenance.
- Provide clear instructions on how fields should be populated, along with.
Data governance tools
Software tools ease the data governance process and automate it, improving speed and efficiency. There are several data governance tools available on the market. Some offer conventional data management capabilities like data lineage monitoring and master data management, while others provide policy and quality management.
Here's a list of the best data governance tools
Ovaledge
Ovaledge is a renowned data governance tool. It performs deep scans of all the locations where data is stored and creates a smart data catalog.
Ovalege will index data regardless of where it is stored in your enterprise ecosystem. Through machine learning, the tool will categorize data through usage statistics, tags, and usernames. It also shows relationships between data assets through manual descriptions and algorithms. With this capability, you can attain a complete understanding of your data even if it lies in a pool of unsorted files.
Ovaledge also lets you create data definitions and data quality standards using a business glossary. The tool enables you to assign responsibilities and roles while at the same time managing access through policy controls. Users also get notifications on data usage and errors.
Collibra
Collibra provides an enterprise-focused data governance system that prioritizes processes and people. Collibra is renowned for its intelligent data governance and management features that provide consistent and accurate data. The tool will allow your enterprise to organize and manage data and manage the rules and policies pertaining to the data.
Collibra also ensures data is kept in a centralized repository, so all users have access to the newest data and policy updates. It is highly effective for cross-functional teams as it facilitates the collaborative process. It allows cooperation between stakeholders to cooperate, build context around the data, and maintain its cleanliness and accuracy.
It gives users interactive data lineage charts (visual representations of the journey data takes from its creation through its transformations over time), and comes with the ability to perform semantic searches, which eases the process of finding specific information. The platform provides details like data policies, flow, and relationships. There are several prebuilt templates to help you quickly get started.
IO-Tahoe
IO-Tahoe is yet another excellent tool for data governance that can optimize the data governance process and help you manage your data. The tool can automatically identify relationships between data assets. It utilizes both known and dark data to provide a comprehensive analysis of the enterprise's data landscape. IO-Tahoe recognizes relationships in structured, semi-structured, and unstructured data forms through complex machine learning algorithms. The tool is also platform-independent, so your organization can use it across various systems with no problems.
Talend
Talend is the ideal tool to help you transition away from data silos and establish governed data hubs. The tool allows you to quickly identify data quality issues and rectify them. Talend provides a unified, end-to-end solution that functions as a single transparent repository for all your organizational data. There's also automated discovery. Besides deep data crawling, the tool can identify and store 80 percent of the information on a specific data asset through machine learning. This helps ensure data is kept up to date.
Talend also uses machine learning to clean the data through validation, smart deduplication, and standardization.
Informatica
Informatica facilitates collaboration between businesses and IT specialists and provides a dedicated enterprise data governance platform that can be used both on-premises and in the cloud. Informatica eliminates data silos and helps security, IT departments, and business teams ensure that data is of high quality and meets compliance requirements. Informatica also enables you to mitigate GPDR risk. With this tool, you can find and protect consumer data regardless of where it lies in your data landscape. It also let s you customer details so you can rest assured you'll be able to reach them if the need arises. Informatica also offers visualization for your data assets.
There are a variety of data governance tools available on the market today. Your ultimate choice will depend entirely on your organization’s needs and data volume.
Note
Remember, you can't merely pick a tool then start your data governance efforts from there. If you want your attempt to succeed, first check that your enterprise is ready. Then, develop a data governance strategy relevant to your organization's needs. After you've done all this, you can now search for a tool that fits your specific requirements.
IBM Data governance solutions
IBM's data governance solutions give you the flexibility to implement any type of data governance strategy. IBM's data governance solution uses machine learning to collect and manage data assets. It jilts you deliver reliable consolidated data resources to users that can be utilized in big data projects. The tool also evaluates data value and helps you find critical data, secure it, and comply with GDPR.
Is your organization ready for Data governance?
You must consider several crucial factors as you evaluate your organization’s maturity and readiness to implement a data governance strategy.
People
People cooperate to identify technological requirements, define processes, and ultimately achieve the data governance outcomes that support strategic drivers. Is your organization committed to data governance? have you formally defined their responsibilities and roles? Do they have the required expertise? Have you designed a change management plan to facilitate organizational alignment?
Processes
Data governance processes enable people to ascertain that your data is appropriately managed across the organization, ensuring that your vital business processes are guided by reliable data. Are your data rules, definitions, and goals relevant and realistic? have you updated your business processes and business rules to ensure a clean, smooth data governance integration?
Contributors
You need IT and business professionals who provide relevant context. Process owners, business leaders, and stewards run both the downstream and upstream processes affected by your initiative, so they must be included in the planning process.
Data governance certifications
If you're interested in gaining an advantage over your rivals and improving your organization’s data governance expertise, Data governance certifications are a worthwhile investment. Certifications evaluate your skills and knowledge against industry and vendor-centric benchmarks to prove that you have the required expertise.
The art of service master digital data management certification
The exam tests knowledge and capabilities ranging from data and project management to IT compliance and business intelligence. The certificate comes in both master and practitioner qualifications based both on exam results and experience.
Data governance and stewardship professional
The data governance and stewardship professional is a certificate offered by the institute for certification of computing professionals. It is a multi-level credential that validates knowledge of data governance. It centers on usability, availability, consistency, data integrity, and security. The certification is offered at the executive management, associate practitioner, principal mastery, data governance professional, and foundation assistant levels.
The enterprise data management
The enterprise data management verified certificate verifies completing the program of the same name delivered through edX, an e-learning and learning platform created by MIT and Harvard. The course, which is designed primarily for business managers, centers on improving the business advantages of functional, reconciled, and big data systems and data assets involved in data management and data analytics at an enterprise level. Focus areas include data profiling principles, master data management, and data integration.
Data is one of an organization’s most vital assets. Data governance helps business leaders and IT departments make sure that data is secure, accessible, and usable. Effective data governance improves data analytics, which in turn improves decision making and operations support. Furthermore, it helps prevent data inconsistencies that may cause integrity issues and several other institutional problems.
Do the first step! Get in touch with BiG EVAL...
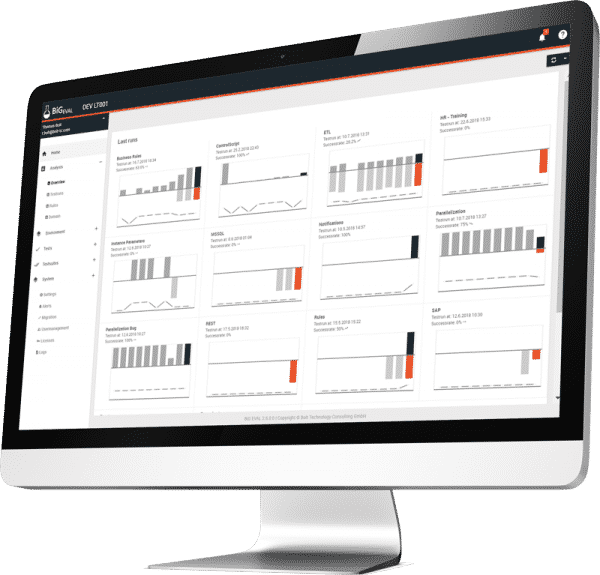
BLOG