DATA QUALITY
Data Quality as a Competitive Advantage: Beyond Compliance
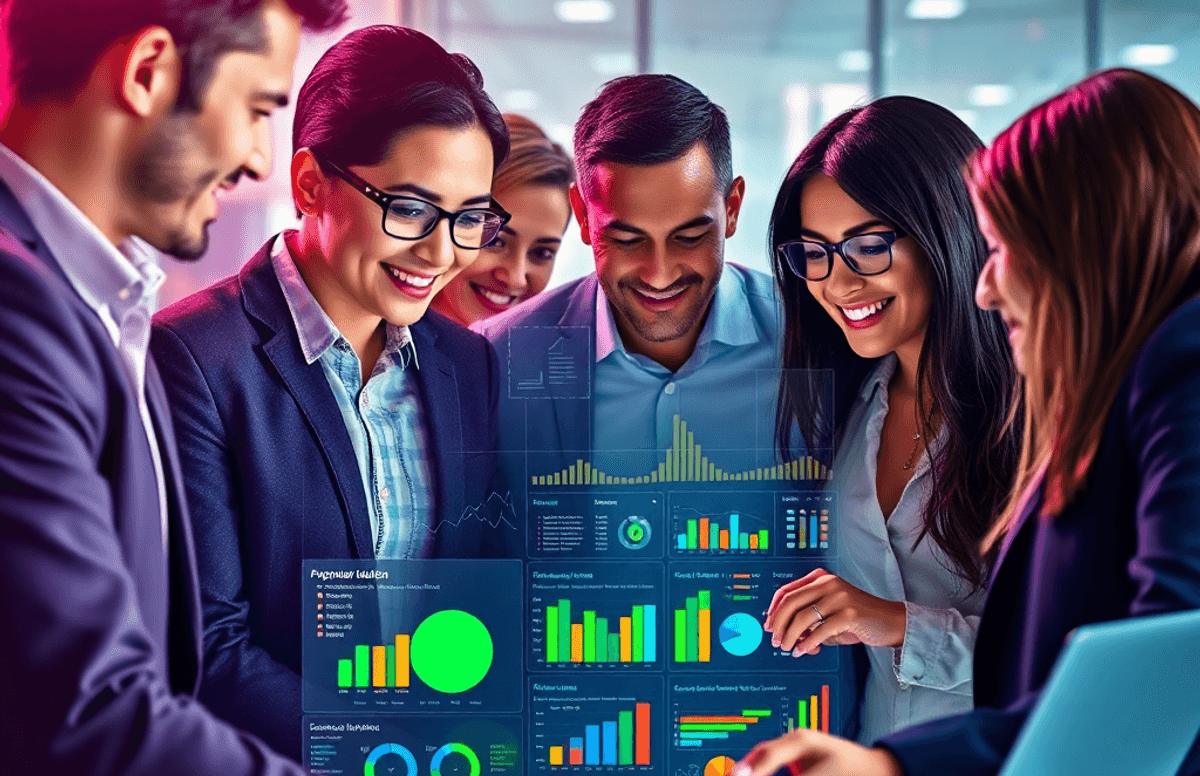
Introduction
Data quality has evolved from being just a compliance requirement to becoming a powerful competitive advantage in today's business world. Organizations now understand that trustworthy data is essential for running successful operations, influencing everything from customer interactions to strategic choices.
High-quality data enables businesses to:
- Create personalized customer experiences
- Make informed strategic decisions
- Drive innovation through reliable insights
- Build lasting customer trust
- Optimize operational efficiency
The shift from viewing data quality as a technical requirement to seeing it as a strategic asset marks a significant evolution in business thinking. Companies that prioritize data reliability gain an advantage over their competitors, establishing themselves as trusted leaders in their industries. This change in how data quality is perceived opens up new opportunities for businesses to use their information resources in ways that create long-lasting competitive advantages.
1. Stand Out in the Market with Reliable Data
In today's competitive world, having reliable data can give you an edge over your rivals. While other companies may still be dealing with inconsistent data, you can use high-quality information to create personalized experiences that make you stand out.
Using Data Insights to Customize Products
Here are some ways you can tailor your products based on what you learn from your data:
- Analyzing customer behavior patterns
- Identifying market gaps and opportunities
- Creating targeted product recommendations
- Developing customized pricing strategies
Real-World Examples of Data-Driven Personalization
Several companies have successfully used reliable data to gain a competitive advantage:
- Netflix: The streaming giant's recommendation engine, powered by analyzing viewing patterns, ratings, and user preferences, drives 80% of viewer activity.
- Spotify: By processing billions of data points, the music streaming service creates unique weekly playlists for each user, resulting in 40+ million followers and higher user retention rates.
- Starbucks: Leveraging its mobile app data, Starbucks understands customer preferences leading to personalized drink recommendations and targeted promotional offers.
These success stories demonstrate how dependable data can lead to concrete benefits in the market - advantages that competitors find hard to duplicate.
2. Building Customer Trust through Data Quality
In today's digital marketplace, data accuracy has a direct impact on customer loyalty. When customers receive personalized recommendations, accurate billing statements, and relevant communications, they develop a deeper trust in your brand.
Key Elements for Building Trust:
- Consistent data accuracy across all interactions
- Clear policies on how data is handled
- Regular updates on how data is being used
- Quick processes for correcting errors
Examples of Transparent Data Practices:
- Apple: Their privacy nutrition labels clearly show users how apps collect and use their data.
- PayPal: Their precise transaction records and protection of sensitive financial data have earned them trust from millions of users worldwide.
Effective Strategies for Building Trust:
- Implement visible processes for validating data
- Provide customers with access to their own data
- Create clear channels for correcting data
- Share regular reports on data quality
Netflix uses accurate viewing data to improve user experiences by recommending content tailored to individual preferences. This approach has resulted in a remarkable 93% customer retention rate, demonstrating that effective management of quality data directly influences customer loyalty.
3. Innovation Enablement via High-Quality Data
High-quality data serves as the foundation for groundbreaking innovation across industries. Companies leveraging clean, accurate data gain predictive insights into emerging market trends and evolving customer preferences.
Data-Driven Innovation Examples:
- Netflix uses viewing patterns to create hit shows like "House of Cards"
- Spotify's Discover Weekly generates personalized playlists from user listening data
- Tesla collects real-time vehicle performance data to enhance autopilot features
Amazon stands out as a prime example of data-driven innovation. The company analyzes billions of customer interactions to:
- Refine product recommendations
- Optimize delivery routes
- Develop new services like Amazon Go stores
Innovation Pipeline Requirements:
- Real-time data collection
- Advanced analytics capabilities
- Machine learning algorithms
- Cross-functional data access
Organizations with robust data quality practices can identify untapped opportunities faster than competitors. This competitive edge enables rapid prototyping and testing of new products based on actual user needs rather than assumptions.
The connection between quality data and innovation extends beyond product development. Companies use reliable datasets to streamline internal processes, create efficient workflows, and build automated systems that free up resources for creative problem-solving.
Moreover, high-quality data is pivotal in the financial sector as well, where it helps in enhancing risk management strategies. By leveraging accurate and timely data, financial institutions can better assess risks, leading to more informed decision-making and ultimately fostering innovation within the industry.
4. Strategic Decision-Making Improvement with Reliable Data
High-quality data transforms executive decision-making from guesswork into a precise science. When leadership teams access trustworthy data, they can spot market trends, identify operational inefficiencies, and capitalize on emerging opportunities with confidence.
Consider Walmart's data-driven inventory management system. By analyzing reliable point-of-sale data, weather patterns, and local events, store managers make accurate stock predictions, reducing waste by 30% and increasing profit margins by 15%.
Key Benefits of Data-Driven Decision Making:
- Reduced risk in strategic planning
- Faster response to market changes
- Improved resource allocation
- Enhanced competitive positioning
Netflix exemplifies the power of quality data in strategic decisions. Their content recommendation engine processes viewer behavior data to inform:
- Content acquisition strategies
- Original programming investments
- Personalized user experiences
The results speak volumes: Netflix maintains a 93% viewer retention rate and saves $1 billion annually through data-driven decision-making in content selection.
Companies like Procter & Gamble use reliable data to optimize their supply chain operations, resulting in 20% cost reduction and 5% increase in customer satisfaction scores.
5. Testing and Validating Data Products for Enhanced Quality Assurance
Testing and validating data products requires a systematic approach to maintain data integrity. Organizations implement comprehensive testing strategies to detect anomalies, inconsistencies, and potential errors before they impact business operations.
Essential Testing Methodologies:
- Unit Testing: Validates individual components of data pipelines
- Integration Testing: Ensures different data systems work together seamlessly
- Performance Testing: Measures data processing speed and system responsiveness
- Load Testing: Evaluates system behavior under peak conditions
Technical Validation Processes:
- Schema Validation: Confirms data structure alignment with predefined formats
- Data Type Verification: Checks for correct formatting of numerical, text, and date fields
- Range Testing: Identifies values outside acceptable parameters
- Completeness Checks: Detects missing or null values
Data validation tools like Great Expectations and Apache Griffin automate these processes, enabling real-time monitoring of data quality metrics. Companies like Netflix implement automated testing frameworks that catch data anomalies before they reach production environments.
Advanced Validation Techniques:
- Pattern Recognition: Identifies unusual data patterns
- Cross-Reference Validation: Verifies data consistency across multiple sources
- Business Rule Validation: Ensures compliance with specific business requirements
- Historical Trend Analysis: Spots deviations from established patterns
These testing and validation practices create a robust foundation for maintaining high-quality data products. Organizations that implement comprehensive testing frameworks experience reduced error rates and improved data reliability.
Implementing Robust Data Governance Frameworks to Sustain Quality Standards
Data governance frameworks are essential for maintaining sustainable data quality management. They provide clear guidelines, responsibilities, and processes to ensure data remains accurate, consistent, and reliable throughout its lifecycle.
Key Components of Effective Data Governance:
1. Data Stewardship Roles
- Chief Data Officer (CDO) oversight
- Dedicated data stewards for each business unit
- Cross-functional data quality teams
2. Metadata Management Policies
- Standardized data definitions
- Documentation requirements
- Version control protocols
- Data lineage tracking
3. Quality Monitoring Procedures
- Real-time data validation checks
- Regular quality audits
- Issue resolution workflows
- Performance metrics tracking
Organizations like Microsoft and IBM implement robust governance frameworks that align with their business objectives. Microsoft's data governance program, for example, includes automated quality checks and clear accountability structures that have reduced data errors by 40%.
Best Practices for Framework Implementation:
- Establish clear data ownership
- Define quality metrics and KPIs
- Create standardized data entry procedures
- Implement automated monitoring tools
- Develop comprehensive documentation
- Regular staff training programs
A well-structured governance framework supports compliance requirements while enabling strategic advantages. Companies like Salesforce demonstrate how strong governance can lead to enhanced customer experiences and operational efficiency through reliable, high-quality data management.
Positioning Data Quality as a Strategic Asset for Long-Term Success
Forward-thinking leaders recognize data quality as a cornerstone of sustainable business growth. Organizations that treat data as a strategic asset gain significant advantages:
- Enhanced Market Intelligence
- Real-time insights into market trends
- Accurate competitor analysis
- Precise customer behavior patterns
- Revenue Growth Opportunities
- Identification of untapped market segments
- Cross-selling and upselling based on reliable customer data
- Reduced costs through efficient resource allocation
Leadership Integration Strategies:
- Establish clear data quality metrics aligned with business objectives
- Invest in advanced analytics capabilities and tools
- Create dedicated data quality teams with direct C-suite reporting lines
- Develop regular data quality assessment protocols
According to research, data-driven organizations consistently outperform their peers by leveraging high-quality data for strategic planning and execution. This competitive edge translates into sustained market leadership and enhanced stakeholder value.
Success Metrics:
- Reduced error rates in reporting
- Increased speed of decision-making
- Higher customer satisfaction scores
- Improved operational efficiency
The importance of data analytics in business cannot be overstated. As we move towards the future, businesses must adapt to become more data-driven enterprises to maintain their competitive edge.
Conclusion
Data quality is a crucial factor in today's competitive business world. Organizations that prioritize data reliability gain significant advantages in several areas:
- Market Leadership: Companies using high-quality data create unique customer experiences, setting themselves apart from competitors.
- Trust Capital: Reliable data practices build long-lasting customer relationships, turning one-time buyers into loyal brand advocates.
- Innovation Engine: Quality data drives ongoing innovation, allowing businesses to anticipate and meet changing market demands.
- Strategic Excellence: Leaders equipped with trustworthy data make confident decisions that lead to organizational success.
To move forward, we need to take proactive steps in managing data quality:
- Implement strict testing protocols
- Establish thorough validation processes
- Maintain strong governance frameworks
- Foster a culture that prioritizes data quality
The future success of your organization depends on how strategically you manage your data assets. Start today by:
- Assessing your current data quality practices
- Identifying weaknesses in your testing and validation methods
- Creating a plan for improving data quality
- Investing in tools and training for your team
Companies that view data quality as a strategic priority will come out on top in their industries. Act now to position your organization as a leader in data-driven excellence.
FAQs (Frequently Asked Questions)
How does data reliability contribute to market differentiation?
Data reliability serves as a key differentiator by enabling organizations to tailor their offerings based on accurate insights. This not only enhances customer experiences but also positions companies ahead of competitors who may lack reliable data.
What strategies can organizations use to build customer trust through data quality?
Organizations can enhance customer trust by ensuring data accuracy and maintaining transparency in their data practices. Implementing clear communication about how data is collected and used, along with showcasing successful examples of reliable data usage, can significantly strengthen customer loyalty.
In what ways does high-quality data enable innovation?
High-quality data allows organizations to anticipate customer needs and derive valuable insights that drive product development and service improvements. Companies like Amazon exemplify this by leveraging customer feedback to foster innovation and enhance their offerings.
Why is trustworthy data crucial for strategic decision-making?
Trustworthy data is essential for informed decision-making as it enhances operational efficiency and responsiveness. Real-world examples show that quality-driven decisions lead to improved outcomes, highlighting the importance of relying on accurate information in executive processes.
What are the best practices for testing and validating data products?
Best practices for testing and validating data products include employing various methodologies such as unit testing, integration testing, and performance testing. Technical validation processes like schema validation, anomaly detection, and consistency checks are critical for identifying discrepancies and ensuring reliability.
How can organizations implement robust data governance frameworks?
Organizations can implement effective data governance frameworks by establishing roles for data stewardship, creating policies for metadata management, and setting procedures for monitoring and resolving quality issues. This comprehensive approach helps maintain high-quality standards throughout the lifecycle of data assets.